Pipeline defect identification method
A defect identification and pipeline technology, applied in the field of computer vision and machine learning, can solve the problems of low classification efficiency and low accuracy, and achieve the effect of improving detection accuracy and detection efficiency, and solving the problem of overlapping defect targets.
- Summary
- Abstract
- Description
- Claims
- Application Information
AI Technical Summary
Problems solved by technology
Method used
Image
Examples
Embodiment Construction
[0037] The technical solutions in the embodiments of the present invention will be clearly and completely described below in conjunction with the accompanying drawings in the embodiments of the present invention. Obviously, the described embodiments are only further detailed descriptions of the present invention rather than all embodiments, and do not constitute Limitations on the Invention.
[0038] The basic idea of DefectNet is to solve target defect detection as a regression problem. Its implementation method is to first obtain a feature map of a certain size through convolutional neuron network feature extraction, and then multi-scale prediction, which divides feature maps of different scales into several networks. Grid, which grid the center of the defect target falls on, which grid is responsible for predicting the defect target. The last is target classification and frame regression. The content of each grid prediction includes 4 coordinate information, a confidence ...
PUM
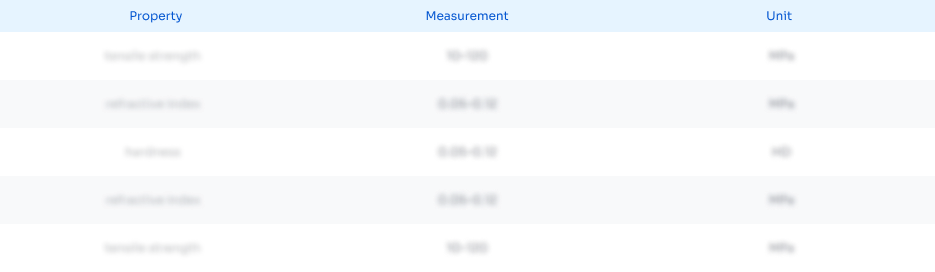
Abstract
Description
Claims
Application Information
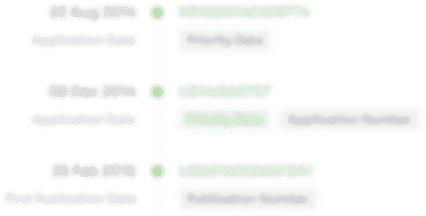
- R&D Engineer
- R&D Manager
- IP Professional
- Industry Leading Data Capabilities
- Powerful AI technology
- Patent DNA Extraction
Browse by: Latest US Patents, China's latest patents, Technical Efficacy Thesaurus, Application Domain, Technology Topic, Popular Technical Reports.
© 2024 PatSnap. All rights reserved.Legal|Privacy policy|Modern Slavery Act Transparency Statement|Sitemap|About US| Contact US: help@patsnap.com