Color fundus image optic cup segmentation method based on deep learning
A fundus image and deep learning technology, applied in the direction of instruments, biological neural network models, character and pattern recognition, etc., can solve the problems of low accuracy of optic cup segmentation and difficulty in meeting large-scale data sets, so as to improve segmentation accuracy and Robust, easy-to-implement, and structurally simple effects
- Summary
- Abstract
- Description
- Claims
- Application Information
AI Technical Summary
Problems solved by technology
Method used
Image
Examples
Embodiment Construction
[0032] Deep learning network structure of the present invention such as figure 1 As shown, Seg-ResNet includes a total of 10 SERes modules, 5 maximum pooling layers, 4 upsampling layers and a loss function layer. The convolution kernel in the network has two sizes, 1×1 and 3×3, and the step size is 1, and each layer of convolution is followed by activation using the ReLU function to increase the nonlinear capability of the network. The convolution size of the max pooling layer is 2×2 with a stride of 2. The SERes in the network structure is a combination module of the residual structure and the channel weighting structure. Each SERes module includes three convolutional layers, and the BatchNorm layer is used in the convolution to perform batch normalization on the data. First, using ResNet as the basic network, the channel weighting structure is used to adjust the response value of each feature channel, and the high-level features are fused with the bottom-level features to f...
PUM
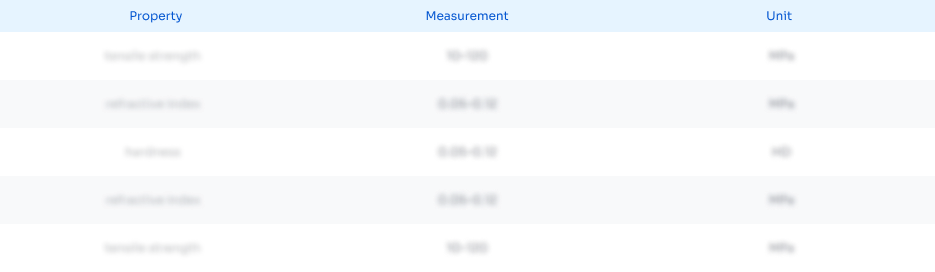
Abstract
Description
Claims
Application Information
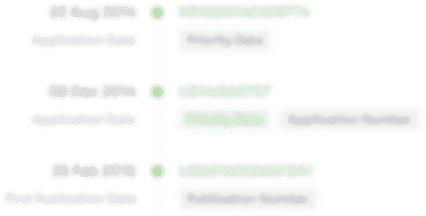
- R&D Engineer
- R&D Manager
- IP Professional
- Industry Leading Data Capabilities
- Powerful AI technology
- Patent DNA Extraction
Browse by: Latest US Patents, China's latest patents, Technical Efficacy Thesaurus, Application Domain, Technology Topic, Popular Technical Reports.
© 2024 PatSnap. All rights reserved.Legal|Privacy policy|Modern Slavery Act Transparency Statement|Sitemap|About US| Contact US: help@patsnap.com