Product quality detection method based on deep neural network
A deep neural network and product quality technology, applied in the field of product quality inspection based on deep neural network, can solve problems such as costing a lot of manpower, material resources, time, and damage, and achieve the effects of simplifying calculation steps, improving detection efficiency, and easy convergence
- Summary
- Abstract
- Description
- Claims
- Application Information
AI Technical Summary
Problems solved by technology
Method used
Image
Examples
Embodiment 1
[0034] A method for product quality detection based on a deep neural network, comprising the following steps:
[0035] S1. Collect sensor data and perform normalization processing to obtain a data matrix:
[0036] First, install the required sensors on the equipment, such as temperature, humidity, vibration, noise, current, voltage, etc. The installed sensors are non-invasive and will not affect the normal production of the product; during the production period, each product produced is numbered , collect sensor data during the production process of this product, and form sensor feature information corresponding to each product. Normalize the data of each sensor of the product. Due to the dimension of each sensor, different sensors use their own dimension for normalization. The formula is as follows:
[0037]
[0038] in It is the real-time data collected by the sensor, with are the maximum and minimum detectable values of this sensor, respectively. For example, ...
PUM
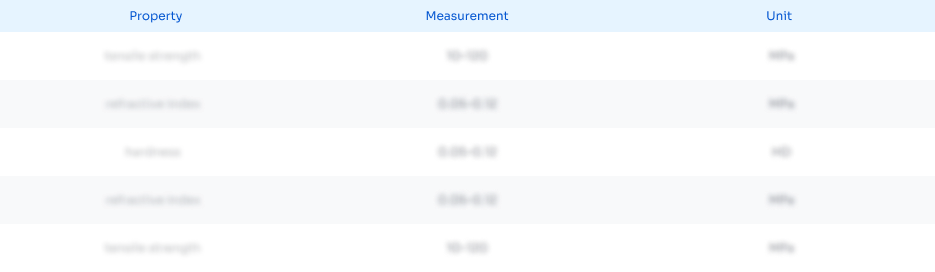
Abstract
Description
Claims
Application Information
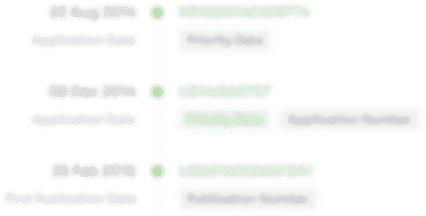
- R&D Engineer
- R&D Manager
- IP Professional
- Industry Leading Data Capabilities
- Powerful AI technology
- Patent DNA Extraction
Browse by: Latest US Patents, China's latest patents, Technical Efficacy Thesaurus, Application Domain, Technology Topic, Popular Technical Reports.
© 2024 PatSnap. All rights reserved.Legal|Privacy policy|Modern Slavery Act Transparency Statement|Sitemap|About US| Contact US: help@patsnap.com