Texture image surface defect detection method based on depth convolution auto-encoder
A self-encoder and deep convolution technology, applied in image enhancement, image analysis, image data processing, etc., can solve the problems of relying on a large number of labeled samples, low versatility and robustness
- Summary
- Abstract
- Description
- Claims
- Application Information
AI Technical Summary
Problems solved by technology
Method used
Image
Examples
Embodiment Construction
[0054] In order to illustrate the purpose and technical solution of the present invention more clearly, the present invention will be further described in detail below in conjunction with the accompanying drawings.
[0055] The embodiment that the inventive method implements is as follows:
[0056] 1) The non-defective texture image is collected, and the Gaussian filter is used to blur each non-defective texture image, and then the processed image is divided into many image blocks with fixed sizes and overlapping parts, and the image blocks are used to build training set and validation set.
[0057] Such as image 3 As shown in the first line, a total of three image sets of different texture primitives are collected, namely Box type, Dot type and Star type; for each texture image, randomly sampled from 25 256×256 defect-free texture images There are 10,000 32×32 image blocks, of which 9,000 are used to form a training set, and 1,000 are used to form a verification set.
[0...
PUM
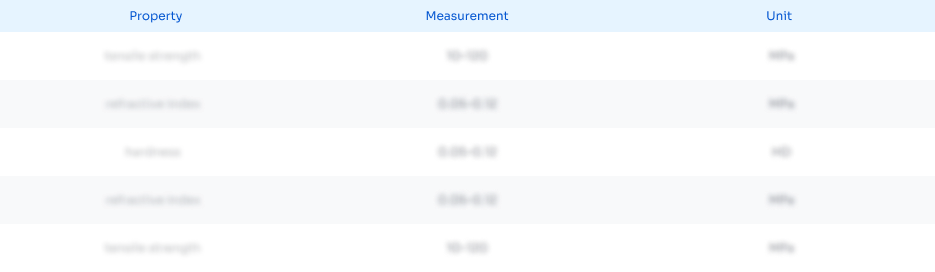
Abstract
Description
Claims
Application Information
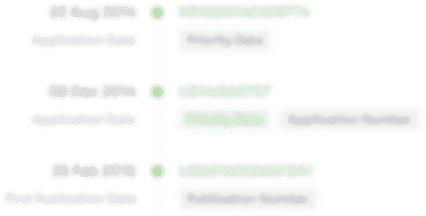
- R&D Engineer
- R&D Manager
- IP Professional
- Industry Leading Data Capabilities
- Powerful AI technology
- Patent DNA Extraction
Browse by: Latest US Patents, China's latest patents, Technical Efficacy Thesaurus, Application Domain, Technology Topic, Popular Technical Reports.
© 2024 PatSnap. All rights reserved.Legal|Privacy policy|Modern Slavery Act Transparency Statement|Sitemap|About US| Contact US: help@patsnap.com