Garbage multi-target detection method based on improved YOLOv3
A detection method and multi-objective technology, applied in the field of artificial intelligence garbage classification and computer vision, can solve problems such as interference clustering, difficult to meet multiple different backgrounds, limitations, etc.
- Summary
- Abstract
- Description
- Claims
- Application Information
AI Technical Summary
Problems solved by technology
Method used
Image
Examples
Embodiment 1
[0040] Embodiment 1: as figure 1 As shown, a garbage multi-target detection method based on improved YOLOv3, the specific steps of the method are as follows:
[0041] Step1, make domestic waste data set and divide it into training data set and test data set;
[0042] Step2. Improve the structure of the traditional YOLOv3 model to obtain an improved YOLOv3 model structure;
[0043] Step3. Based on the improved YOLOv3 model structure, adjust the backbone network depth and densely connected convolution block growth rate K in the improved YOLOv3 model structure, create multiple improved YOLOv3 models, and perform ablation experiments on multiple improved YOLOv3 models to screen out The improved YOLOv3 model with the best performance;
[0044] Step4. Initially set the training parameters of the improved YOLOv3 model with the best performance;
[0045] Step5, then train the improved YOLOv3 model with the best performance: if it is the first training, increase the number of iteratio...
PUM
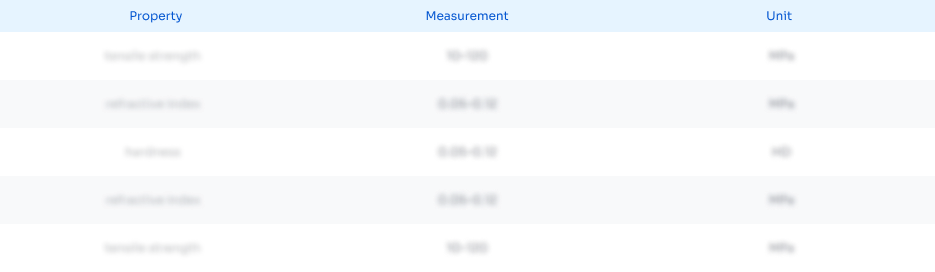
Abstract
Description
Claims
Application Information
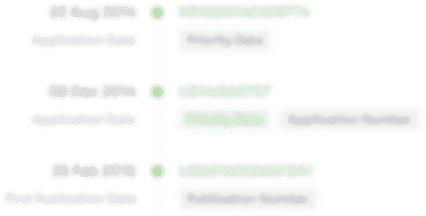
- R&D Engineer
- R&D Manager
- IP Professional
- Industry Leading Data Capabilities
- Powerful AI technology
- Patent DNA Extraction
Browse by: Latest US Patents, China's latest patents, Technical Efficacy Thesaurus, Application Domain, Technology Topic, Popular Technical Reports.
© 2024 PatSnap. All rights reserved.Legal|Privacy policy|Modern Slavery Act Transparency Statement|Sitemap|About US| Contact US: help@patsnap.com