Spatial non-cooperative target relative pose estimation method based on deep learning
A technology of non-cooperative targets and relative poses, which is applied in the field of relative pose estimation of spatial non-cooperative targets based on deep learning. It can solve the problems of being unable to deal with severe occlusions and complex structure of the environment model due to illumination changes, and achieve real-time and autonomy performance, improved model performance and inference estimation, less time-consuming effects
- Summary
- Abstract
- Description
- Claims
- Application Information
AI Technical Summary
Problems solved by technology
Method used
Image
Examples
Embodiment Construction
[0058] The present invention will be further described below in conjunction with the accompanying drawings and examples. It should be understood that the following examples are intended to facilitate the understanding of the present invention, and have no limiting effect on it.
[0059] The invention relates to a method for estimating the relative pose of a space non-cooperative target based on deep learning. The method uses a synthetic image and an image captured by a camera as input, and obtains the position and posture of a space non-cooperative target by designing a convolutional neural network. Multiple space missions including space capture. The present invention mainly includes the following steps: First, considering the lack of public data sets in the current spatial image pose estimation, constructing a three-dimensional model of a non-cooperative target through 3D modeling software, obtaining the data set of the non-cooperative target and dividing it as the training ...
PUM
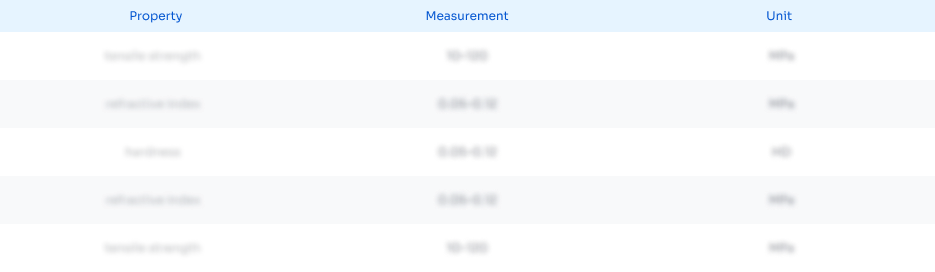
Abstract
Description
Claims
Application Information
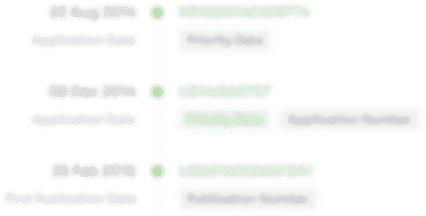
- R&D Engineer
- R&D Manager
- IP Professional
- Industry Leading Data Capabilities
- Powerful AI technology
- Patent DNA Extraction
Browse by: Latest US Patents, China's latest patents, Technical Efficacy Thesaurus, Application Domain, Technology Topic, Popular Technical Reports.
© 2024 PatSnap. All rights reserved.Legal|Privacy policy|Modern Slavery Act Transparency Statement|Sitemap|About US| Contact US: help@patsnap.com