Sequence prediction model training method, prediction system, prediction method and medium
A training method and sequence prediction technology, applied in the field of artificial intelligence, can solve problems such as inability to process asynchronous time series information
- Summary
- Abstract
- Description
- Claims
- Application Information
AI Technical Summary
Problems solved by technology
Method used
Image
Examples
Embodiment 1
[0065] In the present invention, the generated periodic signal is used to train, verify and test the predictive model of the present invention, and to use the tested model to predict, to illustrate the present invention, the model training process is as follows figure 1 shown.
[0066] For randomly encountered asynchronous time series, a periodic signal is generated according to the following formula:
[0067]
[0068] Among them, X t is the value of the signal at time t, N is the number of random periodic signals, α j , ω j ,b j ,β j is the periodic signal parameter of 0-5 random continuous distribution, ε is the standard normal distribution noise, and η is the noise amplification factor. In this example, N=10, η=0.5, and 10,000 groups of sequences with a length of 11 were generated according to the above random formula. Except for the last two time points, the time interval is fixed at 1, and the time interval of other time points in the sequence is 0 -2 random...
Embodiment 2
[0078] In this embodiment, the present invention provides an application in the field of medical treatment to predict and analyze the therapeutic effect of PD-1 inhibitors on patients with lung cancer.
[0079] This example predicts and analyzes the therapeutic effect of PD-1 inhibitors on patients with lung cancer. figure 2 with image 3 The system block diagram shown shows that the training process of the prediction model is as follows:
[0080] Data collection: The basic clinical information of 99 second-line lung cancer patients before and during immunotherapy with PD-1 inhibitors (including patient gender, age, smoking history, tumor family history, radiotherapy history, pathological type, cancer stage), Multiple CT images taken at different times and expert segmentation and labeling (the radiologist marks the main lesion on the CT according to the medical records for accurate segmentation, and is confirmed and marked by a senior oncologist), laboratory test information...
Embodiment 3
[0110] This embodiment is aimed at the short-term prediction of securities prices in secondary market securities transactions, see Figure 4 , the training process of the prediction model is as follows:
[0111] Collect complete securities transaction information in the market within a specified time period, including transaction price, transaction volume, and transaction time as a training set;
[0112] Normalize the collected data, divide the securities transaction price by 50, and divide the transaction volume by 50, and organize them into a time series X sec ∈R T×2 , corresponding to the transaction time point T trade ∈R T . Add feature 1 at the point in time when each transaction price is greater than the average price of n (n∈(5,10,30,60,180)) days in the past, and less than or equal to the new feature 0, to get feature X comp ∈R T×5 , put X sec and x comp Splicing in the feature dimension to get the feature X ts ∈R T×7 .
[0113] input as X ts and T trade ,...
PUM
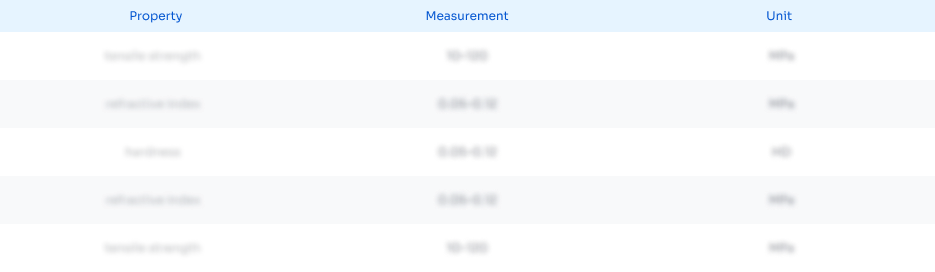
Abstract
Description
Claims
Application Information
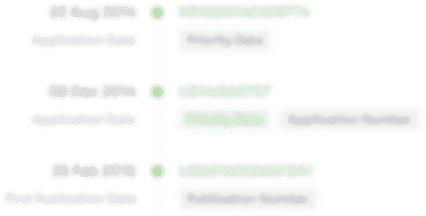
- R&D Engineer
- R&D Manager
- IP Professional
- Industry Leading Data Capabilities
- Powerful AI technology
- Patent DNA Extraction
Browse by: Latest US Patents, China's latest patents, Technical Efficacy Thesaurus, Application Domain, Technology Topic, Popular Technical Reports.
© 2024 PatSnap. All rights reserved.Legal|Privacy policy|Modern Slavery Act Transparency Statement|Sitemap|About US| Contact US: help@patsnap.com