Stable controllable image generation model training method based on W distance
An image generation and model training technology, applied in the field of image recognition, can solve the problems of unstable model training gradient and unstable model gradient descent direction, and achieve the effect of ensuring normal convergence, alleviating mode collapse, and increasing stability.
- Summary
- Abstract
- Description
- Claims
- Application Information
AI Technical Summary
Problems solved by technology
Method used
Image
Examples
Embodiment Construction
[0078] The stable and controllable image generation (CWBLI) model training method based on W (Wasserstein) distance of the present invention comprises the following steps:
[0079] a. Preprocessing the image data to obtain the sample data of the training set and the test set.
[0080] Using the CelebA face dataset as sample data, divide the CelebA face dataset into a training set and a test set. Specifically, 180,000 images can be selected as the training set, and the remaining 22,599 images can be used as the test set. In the original CelebA dataset, each image has 178×218 pixels. In order to highlight the features we need and reduce the complexity of model training, each image in the training set and test set is cropped, such as Figure 11 As shown, the most classic 000001.jpg is taken as an example to show the processing flow, crop out a picture with a fixed face position in each image with a size of 64×64 pixels, and then normalize the cropped picture data.
[0081] b. Co...
PUM
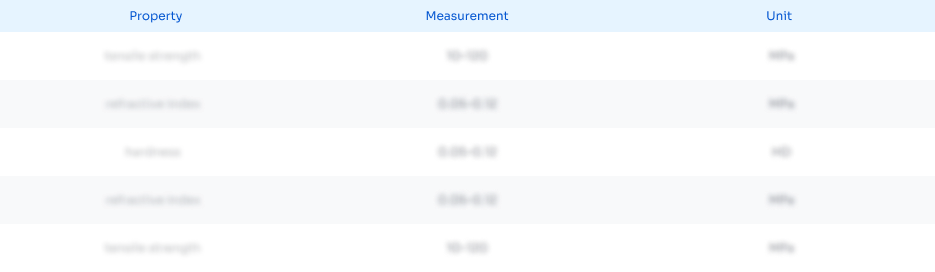
Abstract
Description
Claims
Application Information
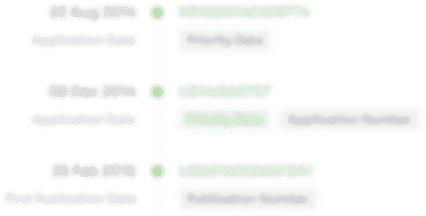
- R&D
- Intellectual Property
- Life Sciences
- Materials
- Tech Scout
- Unparalleled Data Quality
- Higher Quality Content
- 60% Fewer Hallucinations
Browse by: Latest US Patents, China's latest patents, Technical Efficacy Thesaurus, Application Domain, Technology Topic, Popular Technical Reports.
© 2025 PatSnap. All rights reserved.Legal|Privacy policy|Modern Slavery Act Transparency Statement|Sitemap|About US| Contact US: help@patsnap.com