Entity identification method, terminal equipment and storage medium
A technology of entity recognition and entity, which is applied in the field of text recognition, can solve the problems that the accuracy cannot meet expectations, the accuracy of entity boundary determination is insufficient, and the lack of high-quality marked entities is used to achieve the effect of reducing the impact
- Summary
- Abstract
- Description
- Claims
- Application Information
AI Technical Summary
Problems solved by technology
Method used
Image
Examples
Embodiment 1
[0041]The embodiment of the present invention provides an entity identification method, such asfigure 1 withfigure 2 As shown, the method includes the following steps:
[0042]S1: Construct a word graph containing the corresponding domain entities of the text to be recognized.
[0043]In this embodiment, a word graph is constructed for the dictionary in each field, and all the words contained in the dictionary are formed into the vertex set of the word graph. If two characters can form an entity, use the words between the two characters. The straight lines representing the undirected relationship are connected.
[0044]Usually an entity is composed of multiple characters, and the positional relationship of different characters in an entity is different and fixed. In order to obtain the positional relationship of different characters in the entity, the mark set BIESO is set in this embodiment to record different vertices respectively. Which of the following five situations the corresponding d...
Embodiment 2
[0069]The present invention also provides an entity recognition terminal device, including a memory, a processor, and a computer program stored in the memory and running on the processor. The processor implements the implementation of the present invention when the processor executes the computer program. Example 1 of the steps in the above method embodiment.
PUM
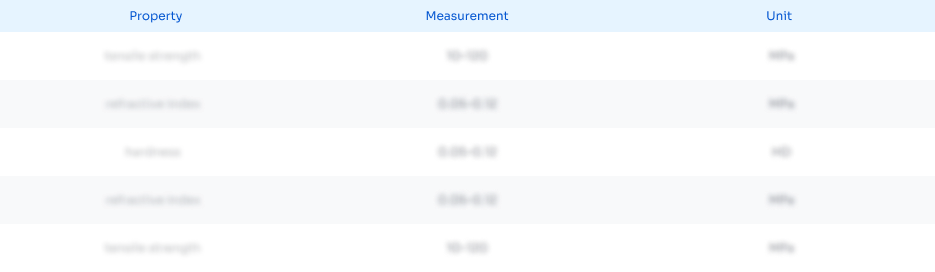
Abstract
Description
Claims
Application Information
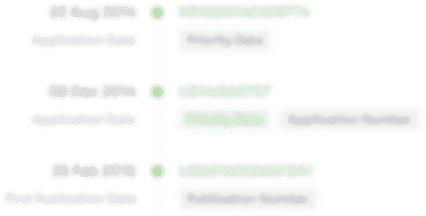
- R&D Engineer
- R&D Manager
- IP Professional
- Industry Leading Data Capabilities
- Powerful AI technology
- Patent DNA Extraction
Browse by: Latest US Patents, China's latest patents, Technical Efficacy Thesaurus, Application Domain, Technology Topic, Popular Technical Reports.
© 2024 PatSnap. All rights reserved.Legal|Privacy policy|Modern Slavery Act Transparency Statement|Sitemap|About US| Contact US: help@patsnap.com