Unsupervised model parameter migration rolling bearing life prediction method
A rolling bearing and life prediction technology, which is applied in neural learning methods, biological neural network models, character and pattern recognition, etc., can solve problems such as difficulty in obtaining label vibration data, large life prediction errors, and difficulty in constructing health indicators
- Summary
- Abstract
- Description
- Claims
- Application Information
AI Technical Summary
Problems solved by technology
Method used
Image
Examples
Embodiment Construction
[0084] For the realization of the present invention, in conjunction with the attached Figures 1 to 12 , elaborated as follows:
[0085] 1 Bottom-up segmentation algorithm theory
[0086] Time series segmentation is the process of decomposing a sequence into subsequences with similar characteristics. The BUP time series segmentation algorithm is a piecewise linear approximation technique, which is completed in two steps of segmentation and merging. First, the time series data points of length T are connected in pairs, divided into non-overlapping T / 2 initial segments, and the fitting cost (fitting error) of merging adjacent segments is calculated. Then, select the minimum fitting cost from it cyclically, if the minimum value is less than the set segment threshold, merge the corresponding two segments, and calculate the fitting cost of the new segment and its preceding and following segments. Repeat this process until all fitting costs are not less than the segmentation thre...
PUM
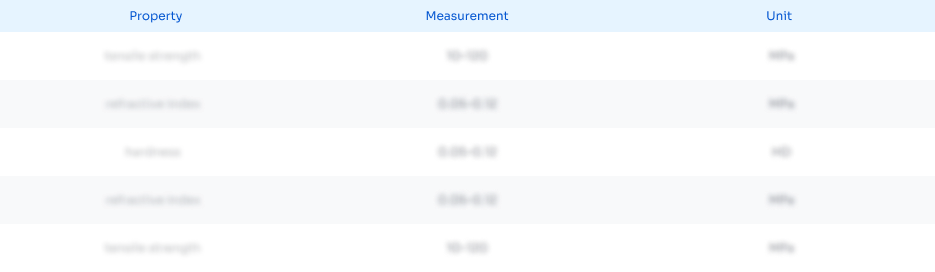
Abstract
Description
Claims
Application Information
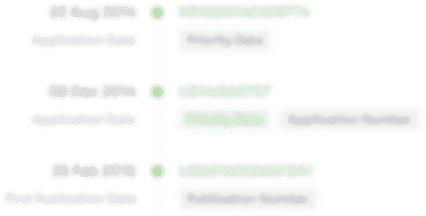
- R&D
- Intellectual Property
- Life Sciences
- Materials
- Tech Scout
- Unparalleled Data Quality
- Higher Quality Content
- 60% Fewer Hallucinations
Browse by: Latest US Patents, China's latest patents, Technical Efficacy Thesaurus, Application Domain, Technology Topic, Popular Technical Reports.
© 2025 PatSnap. All rights reserved.Legal|Privacy policy|Modern Slavery Act Transparency Statement|Sitemap|About US| Contact US: help@patsnap.com