Medical image classification labeling method and system and server
A medical image, manual labeling technology, applied in the medical image classification and labeling method and system, server field, can solve the problems of being easily affected by noise, difficult to ensure the continuity and closure of segmentation edges, etc., to improve processing speed and efficiency. And the effect of accuracy, weight optimization
- Summary
- Abstract
- Description
- Claims
- Application Information
AI Technical Summary
Problems solved by technology
Method used
Image
Examples
Embodiment 1
[0067] see figure 1 , Embodiment 1 discloses a method for classifying and labeling medical images, the method comprising:
[0068] S101: Obtain the original medical image and send it to the manual labeling terminal, and at the same time obtain the data identification code.
[0069] S102: Obtain the manual annotation image (and the corresponding data identification code) returned by the manual annotation terminal, and obtain the pixel boundary of the annotation area through the edge extraction algorithm; wherein, the manual annotation image is manually annotated by the target area of the terminal on the original medical image through artificial Classification and labeling are obtained according to a predetermined strategy; in this embodiment, only the outline of the target area needs to be marked (that is, rough labeling), the work intensity is low, and the labeling speed is very fast. Among them, the pixel boundary is the nearby area where the labeling result can be wrong. ...
Embodiment 2
[0102] see Figure 8 , Embodiment 2 discloses a classification and labeling system for medical images, the system includes:
[0103] User: It is used to upload the original medical image to the server and display the network annotation map.
[0104] Central database: used to obtain and store the original medical images uploaded by users, distribute the original medical images to the server, store the network annotation map and send the network annotation map to the user.
[0105] Server: used to distribute the original medical image to the manual labeling terminal, process the original medical image through the pre-trained full convolutional neural network model to obtain a feature map whose size is the size of the original image, and the depth is the number of labeling types, and obtain the manual labeling map And use the edge extraction algorithm to process the artificially labeled image to obtain the pixel boundary of the labeled area; at the pixel boundary, correct the fe...
Embodiment 3
[0113] see Figure 9 , Embodiment 3 discloses a server, including:
[0114] Data transceiver module: used to receive and store the original medical image sent by the central database, send the original medical image to the manual labeling terminal and send the network labeled map to the central database. is a common structure.
[0115] Image processing module: it is used to process the manually marked image through the edge extraction algorithm to obtain the pixel boundary of the marked area.
[0116] Full convolutional neural network module: used to process the original medical image through the pre-trained full convolutional neural network model to obtain a feature map whose size is the size of the original medical image and whose depth is the number of label types, and the pixel value in the depth direction of the feature map The sequence number of the layer where the largest point is located is the category number of the label.
[0117] Correction module: it is used to ...
PUM
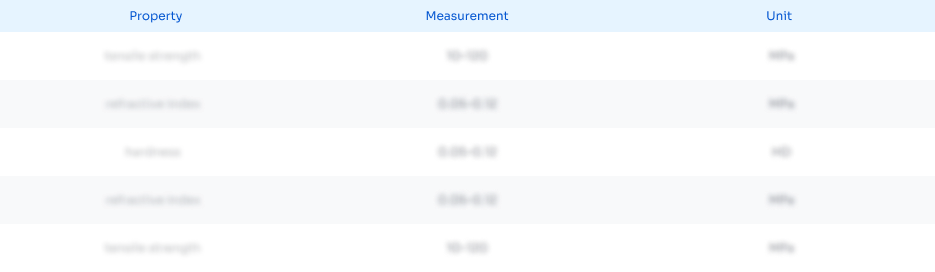
Abstract
Description
Claims
Application Information
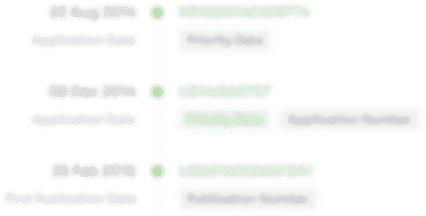
- R&D
- Intellectual Property
- Life Sciences
- Materials
- Tech Scout
- Unparalleled Data Quality
- Higher Quality Content
- 60% Fewer Hallucinations
Browse by: Latest US Patents, China's latest patents, Technical Efficacy Thesaurus, Application Domain, Technology Topic, Popular Technical Reports.
© 2025 PatSnap. All rights reserved.Legal|Privacy policy|Modern Slavery Act Transparency Statement|Sitemap|About US| Contact US: help@patsnap.com