Gaussian embedded neural network model for time sequence prediction and modeling method
A neural network model and neural network technology, applied in the field of Gaussian embedded neural network model and its modeling, can solve the problems of lack of probability information considerations in time series forecasting, and achieve good forecasting results, global excellence, and more global Effect
- Summary
- Abstract
- Description
- Claims
- Application Information
AI Technical Summary
Problems solved by technology
Method used
Image
Examples
Embodiment
[0045] Example: For the Saihanba wind speed raw data set that is sampled every 5 seconds, a new 10-minute sample x is obtained from the average value of 120 5-second samples. Here the step size is 24, by [x i , x i+1 ,...,x i+23 ] get x i+24 , i=1, 2, 3, .... All time series in the dataset are normalized between 0 and 1, and min-max normalization is performed using the MinMaxSscaler of the sklearn package. For the normalized structured data, first random scramble the structured data, and then divide the data into three parts: 81% as the training set to train the prediction model, 9% as the verification set to select the optimal model parameters, The other 10% are used as the test set to evaluate the predictive performance.
[0046] Step 2, long short-term memory neural network layer (LSTM) time series feature extraction:
[0047] The preprocessed data first needs to extract timing information through the LSTM layer. LSTM is chosen as the basic feature extractor because L...
PUM
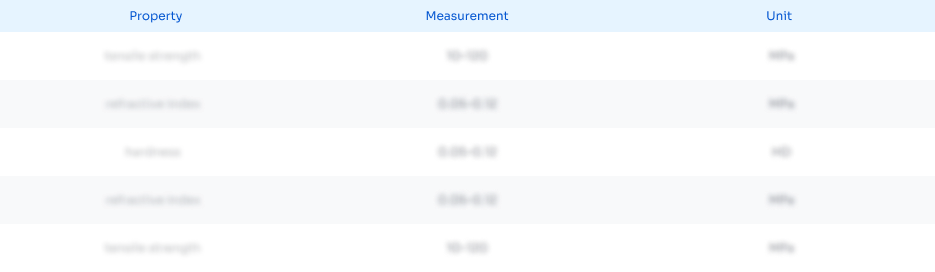
Abstract
Description
Claims
Application Information
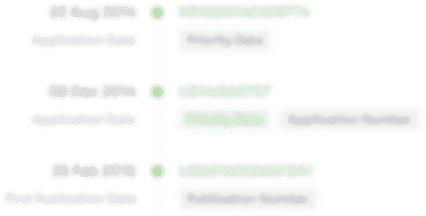
- R&D Engineer
- R&D Manager
- IP Professional
- Industry Leading Data Capabilities
- Powerful AI technology
- Patent DNA Extraction
Browse by: Latest US Patents, China's latest patents, Technical Efficacy Thesaurus, Application Domain, Technology Topic, Popular Technical Reports.
© 2024 PatSnap. All rights reserved.Legal|Privacy policy|Modern Slavery Act Transparency Statement|Sitemap|About US| Contact US: help@patsnap.com