A machine learning-based real-time kpi data anomaly detection method and device
A data anomaly and machine learning technology, applied in the field of intelligent operation and maintenance, can solve problems such as system abnormalities and software bugs, and achieve the effects of guaranteeing service quality, improving operation and maintenance management capabilities, good versatility and practicability
- Summary
- Abstract
- Description
- Claims
- Application Information
AI Technical Summary
Problems solved by technology
Method used
Image
Examples
Embodiment 1
[0058] figure 1 It is a schematic flowchart showing a real-time data anomaly detection method based on machine learning KPI indicators according to an embodiment of the present invention, see figure 1 As shown, the method may include the following steps:
[0059] Online real-time monitoring to obtain KPI data;
[0060] Substituting the KPI data into multiple unsupervised models for screening;
[0061] Putting the data points that are suspiciously abnormal after being screened by all unsupervised models into the first data set;
[0062] Substituting the data points of the first data set into the first detection model for judgment, and storing all unsupervised model screening results as normal and the first detection model judgment result as normal KPI data into the historical database;
[0063] Output judgment result.
Embodiment 2
[0065] figure 2 It is a schematic flowchart showing the establishment of the first detection model of a real-time data anomaly detection method based on machine learning KPI indicators according to an embodiment of the present invention. On the basis of Embodiment 1, this embodiment also has the following content:
[0066] see figure 2 As shown, the establishment of the first detection model in the method may include the following steps:
[0067] Obtain historical monitoring KPI data in the data center operation and maintenance system;
[0068] Perform quality analysis and preprocessing on the acquired KPI data;
[0069] Perform anomaly detection on the preprocessed KPI data according to multiple unsupervised models. When all unsupervised models judge that the current data point is normal, mark the current data point as normal, otherwise mark it as suspected abnormal;
[0070] Establish a training model, and construct an independent forest anomaly detection model (the fir...
Embodiment 3
[0075] image 3 It is a schematic flow diagram showing a data anomaly detection method based on machine learning KPI indicators according to an embodiment of the present invention, see image 3 As shown, on the basis of Embodiment 2, this embodiment also includes the following content:
[0076] Acquisition of KPI data, in chronological order, centrally collects and obtains KPI data monitored by the system.
[0077] The quality analysis and preprocessing of KPI data belong to the data exploration and preparation stage: explore the collected KPI data, better understand its data characteristics, perform quality checks; then preprocess it, that is, convert it into structured data , filter out dirty data, fill missing values and remove noise in KPI data.
[0078] Anomaly detection model training, based on multiple unsupervised models to filter out normal data points in the above preprocessed data, and use the normal data points in these historical data to build an independent f...
PUM
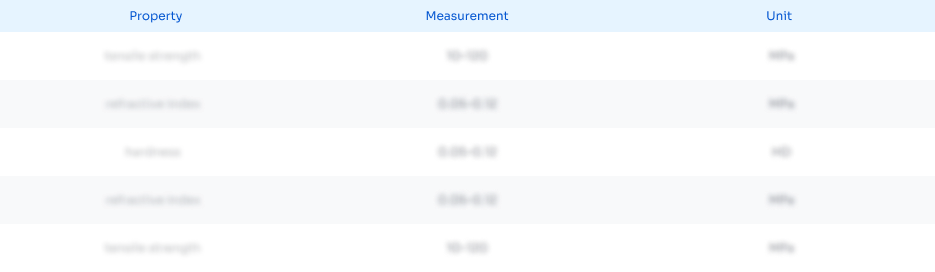
Abstract
Description
Claims
Application Information
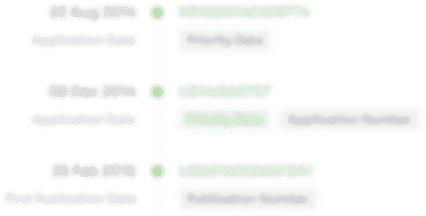
- R&D Engineer
- R&D Manager
- IP Professional
- Industry Leading Data Capabilities
- Powerful AI technology
- Patent DNA Extraction
Browse by: Latest US Patents, China's latest patents, Technical Efficacy Thesaurus, Application Domain, Technology Topic, Popular Technical Reports.
© 2024 PatSnap. All rights reserved.Legal|Privacy policy|Modern Slavery Act Transparency Statement|Sitemap|About US| Contact US: help@patsnap.com