A Construction Method of Weighted Residual Neural Network for Image Classification
A neural network and construction method technology, applied in the field of image classification in computer machine vision, can solve problems such as improving the accuracy of the model, weakening the main branch features, etc., to achieve the effect of improving accuracy, small computational workload, and concise architecture
- Summary
- Abstract
- Description
- Claims
- Application Information
AI Technical Summary
Problems solved by technology
Method used
Image
Examples
Embodiment
[0048] like Figure 1 to Figure 7 As shown, this embodiment provides a method for constructing a weighted residual neural network for image classification, the weighted residual neural network consists of a root module, several weighted incomplete modules and a head module connected in sequence from front to back Composition; Compared with the traditional residual neural network, the weighted residual module proposed in this embodiment replaces the simple and direct addition of the traditional residual module with a weighted summation of the main branch and the bypass branch in the residual module, It effectively avoids the output of the main branch with richer feature information, which is weakened by the output of the bypass branch, thereby improving network performance; in this embodiment, the construction method of the weighted residual neural network includes the following steps
[0049] The first part, the construction of each module
[0050] (1) if Figure 5 As shown,...
PUM
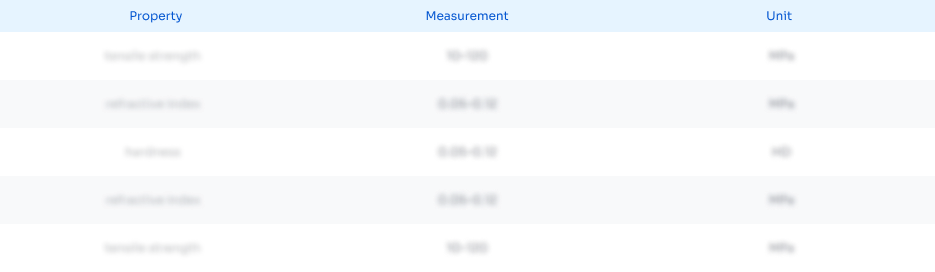
Abstract
Description
Claims
Application Information
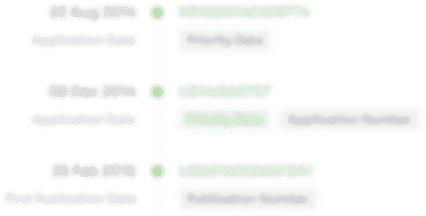
- R&D
- Intellectual Property
- Life Sciences
- Materials
- Tech Scout
- Unparalleled Data Quality
- Higher Quality Content
- 60% Fewer Hallucinations
Browse by: Latest US Patents, China's latest patents, Technical Efficacy Thesaurus, Application Domain, Technology Topic, Popular Technical Reports.
© 2025 PatSnap. All rights reserved.Legal|Privacy policy|Modern Slavery Act Transparency Statement|Sitemap|About US| Contact US: help@patsnap.com