Ultra-short-term load prediction method and system considering data loss and feature redundancy
A load forecasting and data missing technology, applied in forecasting, data processing applications, character and pattern recognition, etc., can solve problems affecting the accuracy of ultra-short-term load forecasting, achieve fairness and breadth, avoid input sequences and output sequences Length limitation, the effect of fast convergence speed
- Summary
- Abstract
- Description
- Claims
- Application Information
AI Technical Summary
Problems solved by technology
Method used
Image
Examples
Embodiment 1
[0025]This embodiment 1 provides an ultra-short-term load forecasting method that considers the lack of time series data and feature redundancy for the lack of data and feature redundancy of multivariate time series data, as well as the low accuracy of existing deep learning load forecasting models. , firstly, by improving the K-Nearest Neighbor (KNN) missing data completion algorithm to process the data sets with missing data; secondly, through the package feature selection method based on the maximum information coefficient (MIC) to obtain super The optimal feature set for short-term load forecasting reduces the feature redundancy of multivariate time-series data; finally, the sequence-to-sequence time-series data processing model improves the model's ability to process time-series information, thereby improving the accuracy of ultra-short-term load forecasting.
[0026] Such as figure 1 As shown, firstly, the data set with missing data problem is processed by improving the ...
Embodiment 2
[0065] In this embodiment 2, on the basis of the embodiment 1, relevant experiments are carried out on the "Individual household electric power consumption Data Set" data set in the UCI database. This data set is a multi-feature time series data set, which describes the electricity consumption information collected by a user from December 2006 to November 2010. The sampling frequency is 1min / time, and the data missing ratio is 1.25%. The present invention The experiment selects the electricity consumption information of 48 collection points every day, and the data contains a total of eight characteristic variables, which are:
[0066] global_active_power: the total active power consumption of the household (kWh);
[0067] global_reactive_power: total household reactive power consumption (kWh);
[0068] voltage: voltage strength (volts);
[0069] global_intensity: current intensity (ampere);
[0070] sub_metering_1: Active energy consumption of the kitchen (Wh);
[0071] su...
PUM
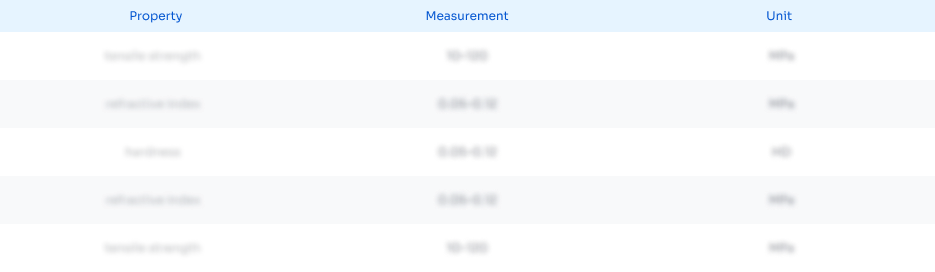
Abstract
Description
Claims
Application Information
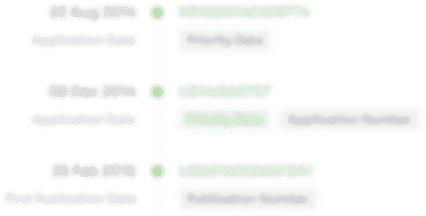
- R&D Engineer
- R&D Manager
- IP Professional
- Industry Leading Data Capabilities
- Powerful AI technology
- Patent DNA Extraction
Browse by: Latest US Patents, China's latest patents, Technical Efficacy Thesaurus, Application Domain, Technology Topic, Popular Technical Reports.
© 2024 PatSnap. All rights reserved.Legal|Privacy policy|Modern Slavery Act Transparency Statement|Sitemap|About US| Contact US: help@patsnap.com