A Soft-Sensor Modeling Method for Debutanizer Tower Based on Aliesn Online Learning Algorithm
A technology of a dealanizer and a modeling method, applied in the field of soft sensing, can solve the problems of complex algorithm, long learning time, and inability to reach fast and real-time
- Summary
- Abstract
- Description
- Claims
- Application Information
AI Technical Summary
Problems solved by technology
Method used
Image
Examples
Embodiment 1
[0065] Such as Figure 1.1 , Figure 1.2 , Figure 1.3 Shown, the present invention proposes a kind of debutanizer tower soft sensor modeling method based on ALIESN online learning algorithm, and the steps are as follows:
[0066] First, use the measuring instruments installed in the butane tower equipment to measure the values of key variables in real time and perform normalized preprocessing, and record them as 7 auxiliary variables xi and 1 leading variable y, where xi is determined by the value of the ith process variable The sample data composition, i ∈ {1, 2, ..., 7} corresponds to the tower top temperature, tower top pressure, reflux flow rate, bottom product outlet flow rate, tray temperature on the 6th layer, tower bottom temperature A and tower bottom temperature B , and then use the NARX model to model 7 auxiliary variables and 1 leading variable as the input variables of ALIESN;
[0067] Secondly, for the first n data of ALIESN input variables, use the ALIESN r...
Embodiment 2
[0071] Such as Figure 2.1 , Figure 2.2 , Figure 2.3 As shown, using the same conditions as in Example 1 for learning, the mean square error value is 1.466215709364227*10 -5 .
Embodiment 3
[0073] Such as Figure 3.1 , Figure 3.2 , Figure 3.3 As shown, using the same conditions as in Examples 1 and 2 for learning, the mean square error value is 2.757009214241490*10 -5 .
PUM
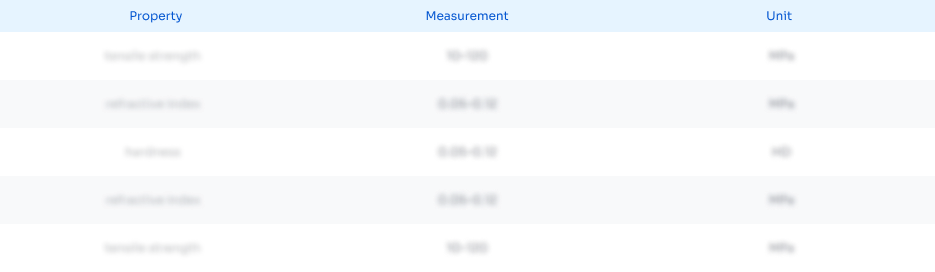
Abstract
Description
Claims
Application Information
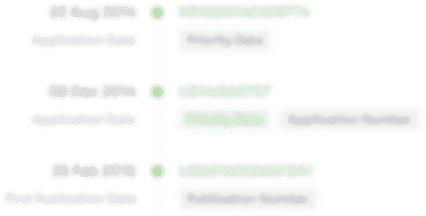
- R&D Engineer
- R&D Manager
- IP Professional
- Industry Leading Data Capabilities
- Powerful AI technology
- Patent DNA Extraction
Browse by: Latest US Patents, China's latest patents, Technical Efficacy Thesaurus, Application Domain, Technology Topic, Popular Technical Reports.
© 2024 PatSnap. All rights reserved.Legal|Privacy policy|Modern Slavery Act Transparency Statement|Sitemap|About US| Contact US: help@patsnap.com