Power distribution network fault diagnosis method based on deep feature clustering and LSTM
A distribution network fault and deep feature technology, which is applied in character and pattern recognition, biological neural network models, instruments, etc., can solve problems such as data imbalance, difficult training, and tediousness, so as to reduce negative impacts, avoid loss, and improve The effect of accuracy
- Summary
- Abstract
- Description
- Claims
- Application Information
AI Technical Summary
Problems solved by technology
Method used
Image
Examples
Embodiment Construction
[0049] The accompanying drawings are for illustrative purposes only, and should not be construed as limiting the present invention; in order to better illustrate this embodiment, certain components in the accompanying drawings will be omitted, enlarged or reduced, and do not represent the size of the actual product; for those skilled in the art It is understandable that some well-known structures and descriptions thereof may be omitted in the drawings. The positional relationship described in the drawings is for illustrative purposes only, and should not be construed as limiting the present invention.
[0050] Such as figure 1 As shown, a distribution network fault diagnosis method based on deep feature clustering and LSTM includes the following steps:
[0051] S1. Obtain typical data sets under various faults in the distribution network to form a fault sample data set, and divide the fault sample data set into a labeled sample set and an unlabeled sample set according to whe...
PUM
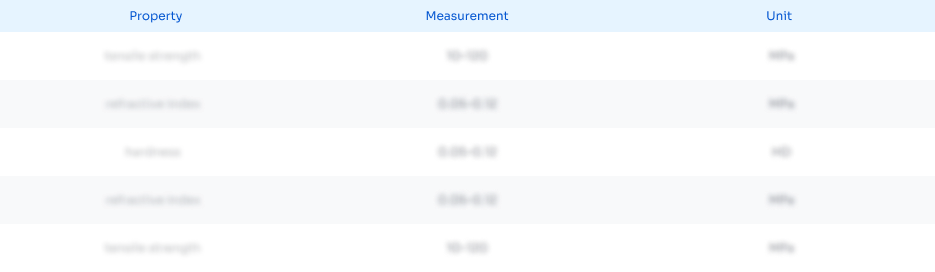
Abstract
Description
Claims
Application Information
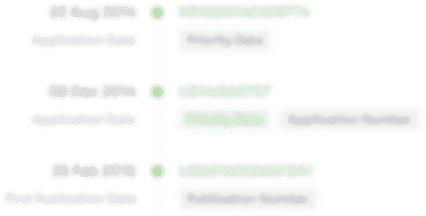
- R&D
- Intellectual Property
- Life Sciences
- Materials
- Tech Scout
- Unparalleled Data Quality
- Higher Quality Content
- 60% Fewer Hallucinations
Browse by: Latest US Patents, China's latest patents, Technical Efficacy Thesaurus, Application Domain, Technology Topic, Popular Technical Reports.
© 2025 PatSnap. All rights reserved.Legal|Privacy policy|Modern Slavery Act Transparency Statement|Sitemap|About US| Contact US: help@patsnap.com