Sparse self-representation subspace clustering algorithm for self-adaptive local structure embedding
A local structure and clustering algorithm technology, applied in the information field, can solve the problems of sensitive adjacency graph quality, hindering the construction of high-quality graphs, etc., and achieve the effect of stable performance
- Summary
- Abstract
- Description
- Claims
- Application Information
AI Technical Summary
Problems solved by technology
Method used
Image
Examples
specific Embodiment
[0098] figure 2 Is this embodiment and other subspace clustering algorithms, namely PCA-K-means clustering, structured optimal graph feature selection (SOGFS), unsupervised discriminative feature selection (UDFS), sparse subspace clustering (SSC) Compared with the clustering accuracy curves of projection clustering (PCAN) with adaptive nearest neighbors (PCAN) under different numbers of selected features; it can be found that as the selected features increase, the clustering results become better; this trend It shows that the intrinsic structure of MSRA25 dataset is embedded in high-dimensional space; SSS outperforms other subspace clustering methods when it learns local structure from input space; image 3 As can be seen in , this example achieves good separation results on the wine dataset, which directly verifies that SSS learns a discriminative structure in the subspace; Figure 4 shows the process of graph S learning adjacency graph A, Figure 4 The non-spherical synth...
PUM
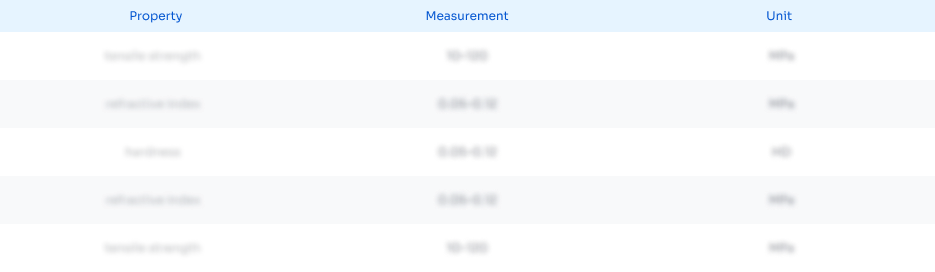
Abstract
Description
Claims
Application Information
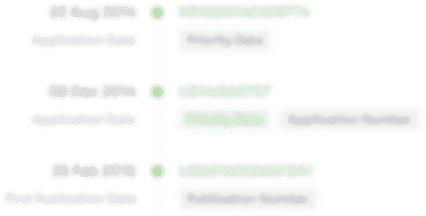
- R&D
- Intellectual Property
- Life Sciences
- Materials
- Tech Scout
- Unparalleled Data Quality
- Higher Quality Content
- 60% Fewer Hallucinations
Browse by: Latest US Patents, China's latest patents, Technical Efficacy Thesaurus, Application Domain, Technology Topic, Popular Technical Reports.
© 2025 PatSnap. All rights reserved.Legal|Privacy policy|Modern Slavery Act Transparency Statement|Sitemap|About US| Contact US: help@patsnap.com