Micro-surface material reconstruction method and system based on deep learning
A deep learning and micro-surface technology, applied in 3D image processing, image analysis, image enhancement, etc., can solve problems such as inability to use practical applications, low resolution of material maps, time-consuming and labor-intensive data sets, etc., to reduce the amount of network parameters. , shorten the training time, and ensure the effect of quality
- Summary
- Abstract
- Description
- Claims
- Application Information
AI Technical Summary
Problems solved by technology
Method used
Image
Examples
Embodiment 1
[0039] This embodiment provides a micro-surface material reconstruction method based on deep learning;
[0040] A micro-surface material reconstruction method based on deep learning, including:
[0041] S101: Acquire a captured image of a real-world material;
[0042] S102: Input the captured image of the material into the network framework for material reconstruction and synthesis after training, and the generator network in the network framework for material reconstruction and synthesis outputs a diffuse reflection map, a reflection map, a roughness map and a normal map;
[0043] S103: Draw the diffuse reflection map, the reflection map, the roughness map and the normal map into a rendering image.
[0044] As one or more embodiments, after acquiring the captured image of the real-world material, before the step of inputting the captured image of the material into the pre-training generator network, it further includes: performing gamma correction and summing on the captured...
Embodiment 2
[0130] Embodiment 2, this embodiment provides a micro-surface material reconstruction system based on deep learning;
[0131] Embodiment two
[0132] This embodiment provides a micro-surface material reconstruction system based on deep learning;
[0133] Micro-surface material reconstruction system based on deep learning, including:
[0134] a data generation module configured to: acquire captured images of real-world materials;
[0135] The material reconstruction module is configured to: input the captured image of the material into the pre-training generator network, and output a diffuse reflection map, a reflection map, a roughness map and a normal map;
[0136] The drawing module is configured to: draw a rendering image from the diffuse reflection map, the reflection map, the roughness map and the normal map.
[0137] It should be noted here that the above-mentioned data generation module, material reconstruction module and drawing module correspond to Step S101 to Ste...
Embodiment 3
[0141] This embodiment also provides an electronic device, including: one or more processors, one or more memories, and one or more computer programs; wherein, the processor is connected to the memory, and the one or more computer programs are programmed Stored in the memory, when the electronic device is running, the processor executes one or more computer programs stored in the memory, so that the electronic device executes the method described in Embodiment 1 above.
[0142] It should be understood that in this embodiment, the processor can be a central processing unit CPU, and the processor can also be other general-purpose processors, digital signal processors DSP, application specific integrated circuits ASIC, off-the-shelf programmable gate array FPGA or other programmable logic devices , discrete gate or transistor logic devices, discrete hardware components, etc. A general-purpose processor may be a microprocessor, or the processor may be any conventional processor, o...
PUM
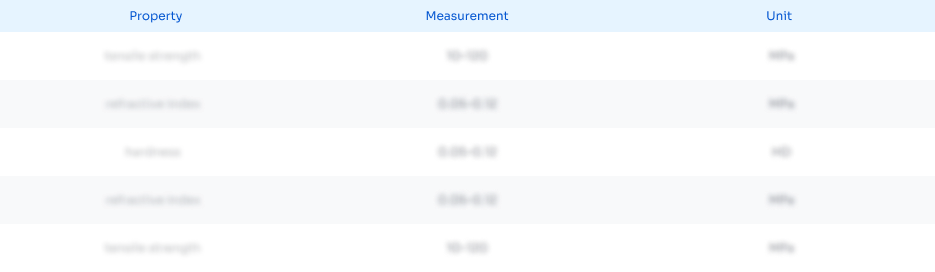
Abstract
Description
Claims
Application Information
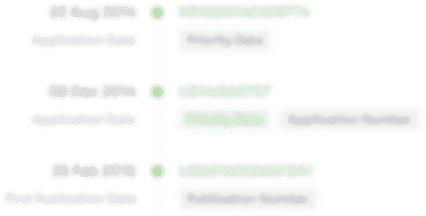
- R&D Engineer
- R&D Manager
- IP Professional
- Industry Leading Data Capabilities
- Powerful AI technology
- Patent DNA Extraction
Browse by: Latest US Patents, China's latest patents, Technical Efficacy Thesaurus, Application Domain, Technology Topic, Popular Technical Reports.
© 2024 PatSnap. All rights reserved.Legal|Privacy policy|Modern Slavery Act Transparency Statement|Sitemap|About US| Contact US: help@patsnap.com