System power consumption optimized lightweight system and method with autonomous learning function
A technology of system power consumption and self-learning, which is applied in the field of deep learning, can solve the problems of not being able to realize the real-time performance of tasks well, and achieve the effect of realizing intelligence, improving efficiency and reducing power consumption
- Summary
- Abstract
- Description
- Claims
- Application Information
AI Technical Summary
Problems solved by technology
Method used
Image
Examples
Embodiment 1
[0030] Such as figure 1 As shown, a lightweight system with self-learning function and optimized system power consumption, the system includes: a data acquisition unit configured to collect real-time operating data of each operating unit in the system; the real-time operating data includes at least: each The temperature data, current data, voltage data and running time of the running unit; the sample state estimation unit is configured to estimate the probability of the collected real-time running data based on the collected real-time running data, and establish a state sample set; The judgment unit is configured to use the established state judgment function based on the established state sample set to judge the operating state of each operating unit in the current system, and obtain whether each operating unit is in a concave curve operating state or a convex curve operating state; The control unit is configured to use the operating state of each operating unit obtained base...
Embodiment 2
[0034] On the basis of the previous embodiment, the sample state estimation unit, based on the collected real-time operation data, performs probability estimation on the collected real-time operation data, and the method for establishing a state sample set performs the following steps: the collected real-time operation data Run the data, when the time is 0, that is, when k is 0, according to the probability distribution, the initial state sample set is established: s, w, Q and P represent the degree data, current data, voltage data and running time respectively; get the weight: Among them, N is the total number of real-time running data; then calculate the instantaneous value at a certain moment: let k=k+1, calculate the instantaneous value at k+1 moment; use the following formula to summarize the peak value of the instantaneous value: Among them, K k is the predicted value of the wave at the kth moment; Indicates the real instantaneous value; z k Indicates the es...
Embodiment 3
[0037] On the basis of the previous embodiment, the state judging unit, based on the established state sample set, uses the established state judgment function to judge the operating state of each operating unit in the current system, and obtains whether each operating unit is out of a concave curve The method of running state or convex curve running state performs the following steps: Use the following formula as the state judgment function: Power min =Min∑ i∈N [s c (i)·w c +Q l (i)·p l +P i (i)·p i ]+∑ i,j∈N,i≠j t(i,j)·p t ; Among them, Power min is the calculated power consumption, N is the total number of real-time running data, c is the first adjusted square number, the value range is: 1~3, l is the second adjusted square number, the value range is 2~4 , p is the adjustment probability, the value range is: 25% to 75%, t is the curve time variable; the data set corresponding to each unit in the state sample set is input into the state judgment function as an input...
PUM
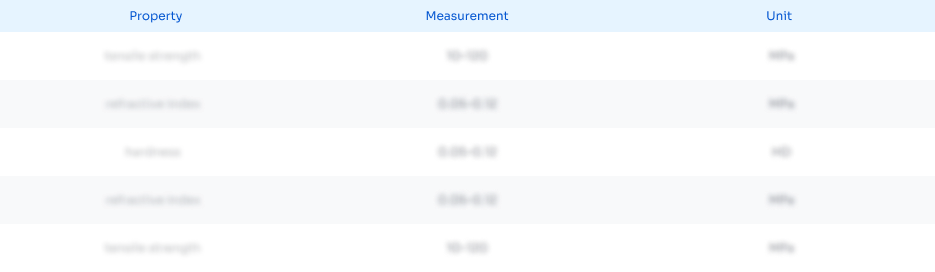
Abstract
Description
Claims
Application Information
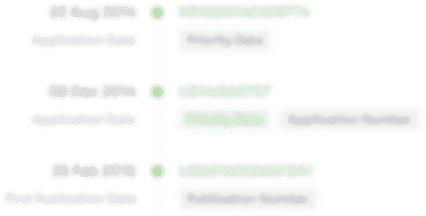
- R&D
- Intellectual Property
- Life Sciences
- Materials
- Tech Scout
- Unparalleled Data Quality
- Higher Quality Content
- 60% Fewer Hallucinations
Browse by: Latest US Patents, China's latest patents, Technical Efficacy Thesaurus, Application Domain, Technology Topic, Popular Technical Reports.
© 2025 PatSnap. All rights reserved.Legal|Privacy policy|Modern Slavery Act Transparency Statement|Sitemap|About US| Contact US: help@patsnap.com