Mechanical intelligent fault prediction method based on automatic convolutional neural network
A convolutional neural network and fault prediction technology, applied in the direction of neural learning methods, biological neural network models, kernel methods, etc., can solve the problem of reducing the prediction accuracy and generalization ability of fault diagnosis models, affecting the effect of fault feature extraction, and consuming a lot of labor Energy and other issues to achieve the effect of reducing the dependence on manual experience, reducing the impact of training effects, and improving the effect
- Summary
- Abstract
- Description
- Claims
- Application Information
AI Technical Summary
Problems solved by technology
Method used
Image
Examples
Embodiment Construction
[0043] In order to make the purpose, technical solution and advantages of the present invention clearer, the embodiments of the present invention will be further described below in conjunction with the accompanying drawings.
[0044] Please refer to figure 1 , an automatic convolutional neural network-based fault prediction method for machine intelligence, including the following:
[0045] S101: Acquire equipment fault signals, and perform preprocessing on them, to obtain preprocessed fault signals;
[0046] In the embodiment of the present invention, the collected equipment fault signals are mainly vibration signals, the time-series vibration signals are intercepted from the collected signal segments, and the time-frequency analysis is performed on the vibration signals by using the S-transform method, and the obtained time-frequency diagram is 2D matrix. Make the time-frequency graph into a 224*224-dimensional size, that is, to realize the preprocessing of the equipment f...
PUM
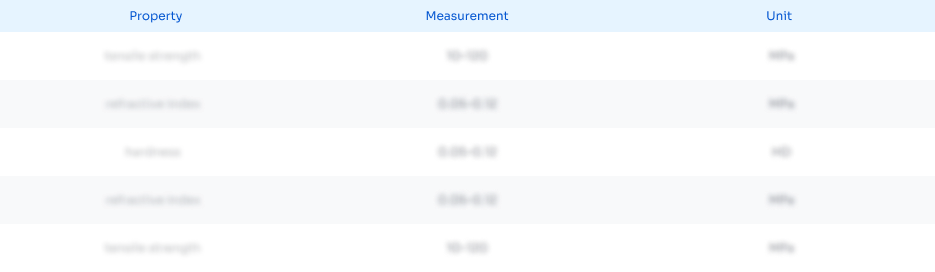
Abstract
Description
Claims
Application Information
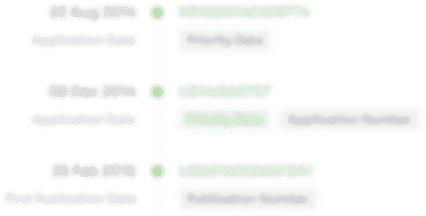
- R&D Engineer
- R&D Manager
- IP Professional
- Industry Leading Data Capabilities
- Powerful AI technology
- Patent DNA Extraction
Browse by: Latest US Patents, China's latest patents, Technical Efficacy Thesaurus, Application Domain, Technology Topic, Popular Technical Reports.
© 2024 PatSnap. All rights reserved.Legal|Privacy policy|Modern Slavery Act Transparency Statement|Sitemap|About US| Contact US: help@patsnap.com