Parameter estimation model training method and device, equipment and storage medium
A technology for estimating models and training methods, which is applied in the field of image processing, can solve the problems that the estimation accuracy of reconstruction parameters cannot be guaranteed, the operation of 3D face reconstruction is cumbersome and complex, and the acquisition of reconstruction parameters requires a lot, so as to improve the estimation accuracy and deformation process. Accurate, the effect of ensuring accuracy
- Summary
- Abstract
- Description
- Claims
- Application Information
AI Technical Summary
Problems solved by technology
Method used
Image
Examples
Embodiment 1
[0034] Figure 1A It is a flow chart of a method for training a parameter estimation model provided by Embodiment 1 of the present invention. This embodiment can be applied to any situation of 3D face reconstruction that requires estimation of corresponding reconstruction parameters. The training method of the parameter estimation model provided in this embodiment can be executed by the training device of the parameter estimation model provided in the embodiment of the present invention, which can be realized by means of software and / or hardware, and integrated in the computer equipment executing the method middle.
[0035] Specifically, refer to Figure 1A , the method may include the following steps:
[0036] S110, input each training sample in the face image training set into a pre-built neural network model, estimate the reconstruction parameters specified for 3D face reconstruction, and input the reconstruction parameters into the pre-built 3D deformation model, reconstru...
Embodiment 2
[0055] Figure 2A It is a flow chart of a method for training a parameter estimation model provided in Embodiment 2 of the present invention, Figure 2B It is a schematic structural diagram of a 3D deformable model used for 3D face reconstruction in the method provided in Embodiment 2 of the present invention. This embodiment is optimized on the basis of the foregoing embodiments.
[0056] It should be noted that the three-dimensional deformation model in this embodiment is composed of a dual principal component analysis (Principal Component Analysis, PCA) model and a single PCA model, such as Figure 2B As shown, the double PCA model is mainly used to model the change of face shape and expression in the process of 3D face reconstruction, while the single PCA model is mainly used to model the change of face albedo in the process of 3D face reconstruction. modeling.
[0057] Wherein, the double PCA model in the present embodiment can define the three-dimensional average huma...
Embodiment 3
[0077] Figure 3A It is a flowchart of a method for training a parameter estimation model provided by Embodiment 3 of the present invention, Figure 3B It is a schematic diagram of the principle of the training process of the parameter estimation model provided by Embodiment 3 of the present invention. This embodiment is optimized on the basis of the foregoing embodiments. Specifically, such as Figure 3A As shown, the loss function under multiple two-dimensional supervision information in this embodiment may include: image pixel loss function, key point loss function, identity feature loss function, albedo penalty function, and the target in the reconstruction parameters specified by three-dimensional face reconstruction Reconstruct the regular term corresponding to the parameters. At this time, it mainly explains in detail the specific setting method of the loss function under the multiple two-dimensional supervision information referenced by the parameter estimation model...
PUM
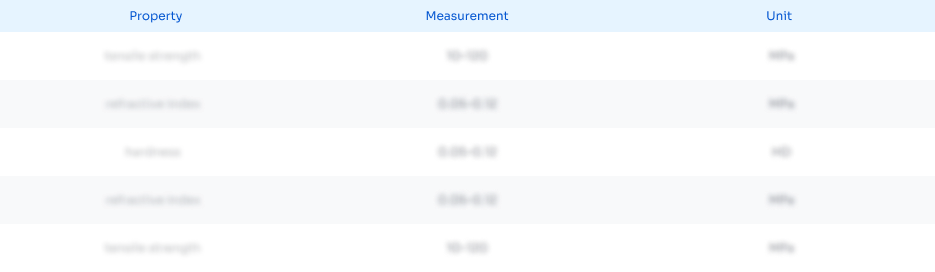
Abstract
Description
Claims
Application Information
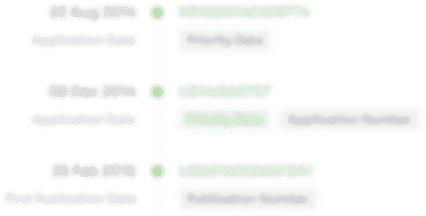
- R&D Engineer
- R&D Manager
- IP Professional
- Industry Leading Data Capabilities
- Powerful AI technology
- Patent DNA Extraction
Browse by: Latest US Patents, China's latest patents, Technical Efficacy Thesaurus, Application Domain, Technology Topic, Popular Technical Reports.
© 2024 PatSnap. All rights reserved.Legal|Privacy policy|Modern Slavery Act Transparency Statement|Sitemap|About US| Contact US: help@patsnap.com