Rotary machine fault diagnosis method based on improved convolutional neural network
A technology of convolutional neural network and rotating machinery, applied in biological neural network models, neural architecture, geometric CAD, etc., can solve the problems of easy error deletion of small fault features, large subjectivity and blindness, noise masking, etc., to reduce training Flexible and convenient monitoring of parameters, calculation time, and operating status, and the effect of improving diagnostic speed and efficiency
- Summary
- Abstract
- Description
- Claims
- Application Information
AI Technical Summary
Problems solved by technology
Method used
Image
Examples
Embodiment Construction
[0025] In order to better understand the present invention, the present invention will be further described below in conjunction with the accompanying drawings and embodiments. It should be understood that the specific embodiments described here are only used to explain the present invention, not to limit the present invention. In addition, the technical features involved in the various embodiments of the present invention described below can be combined with each other as long as they do not constitute a conflict with each other.
[0026] Please refer to the attached figure 1 ~ attached Figure 5 , the preferred embodiment of the present invention provides a method for diagnosing rotating machinery faults based on an improved convolutional neural network, which includes steps 1 to 7.
[0027] Step 1: Acquiring one-dimensional time series fault vibration signals of rotating machinery. Specifically, a vibration acceleration sensor is installed on the rotating machine, and th...
PUM
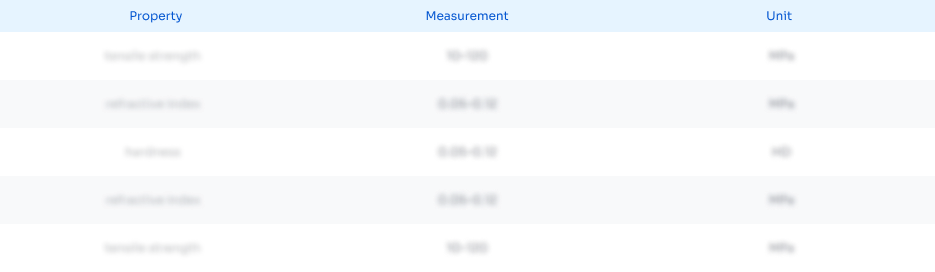
Abstract
Description
Claims
Application Information
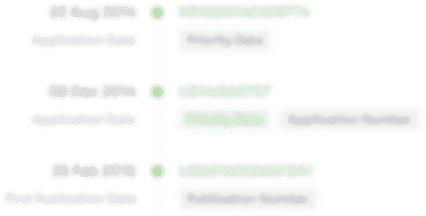
- R&D Engineer
- R&D Manager
- IP Professional
- Industry Leading Data Capabilities
- Powerful AI technology
- Patent DNA Extraction
Browse by: Latest US Patents, China's latest patents, Technical Efficacy Thesaurus, Application Domain, Technology Topic, Popular Technical Reports.
© 2024 PatSnap. All rights reserved.Legal|Privacy policy|Modern Slavery Act Transparency Statement|Sitemap|About US| Contact US: help@patsnap.com