Lightweight semantic segmentation method based on multi-scale visual feature extraction
A multi-scale feature and visual feature technology, applied in neural learning methods, image analysis, image data processing, etc., can solve the problems of large semantic segmentation network model and slow reasoning speed
- Summary
- Abstract
- Description
- Claims
- Application Information
AI Technical Summary
Problems solved by technology
Method used
Image
Examples
Embodiment Construction
[0054] The present invention will be described in detail below in conjunction with the accompanying drawings and specific embodiments.
[0055] The present invention provides a lightweight semantic segmentation method based on multi-scale visual feature extraction, such as figure 1 As shown, the specific steps are as follows:
[0056] Step 1. Construct a lightweight convolutional neural network LitNet based on multi-scale feature extraction, extract image features through a feature extractor, pass the features into the spatial pyramid module of fusion hole convolution to extract image multi-scale features, and finally through simple upsampling The module completes feature integration and restores image resolution;
[0057]Its network structure is divided into 3 modules: 1) feature extraction module; 2) multi-scale fusion module; 3) upsampling module;
[0058] After the image is input into the network, it first performs down-sampling to extract features through the feature ex...
PUM
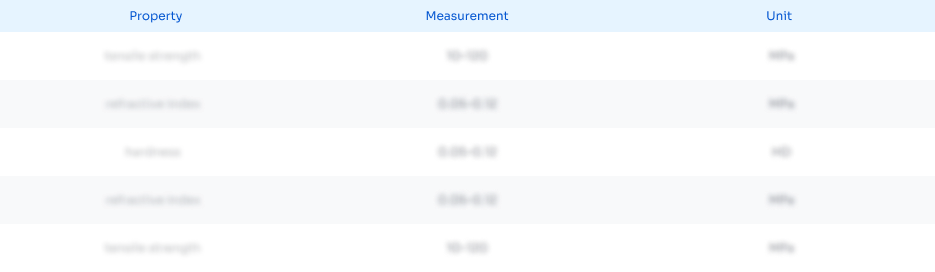
Abstract
Description
Claims
Application Information
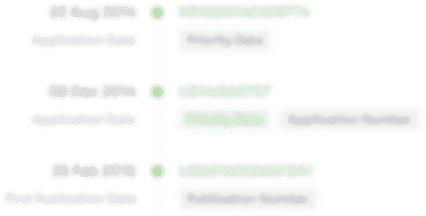
- R&D
- Intellectual Property
- Life Sciences
- Materials
- Tech Scout
- Unparalleled Data Quality
- Higher Quality Content
- 60% Fewer Hallucinations
Browse by: Latest US Patents, China's latest patents, Technical Efficacy Thesaurus, Application Domain, Technology Topic, Popular Technical Reports.
© 2025 PatSnap. All rights reserved.Legal|Privacy policy|Modern Slavery Act Transparency Statement|Sitemap|About US| Contact US: help@patsnap.com