Electroencephalogram signal emotion recognition method based on dimension model
A technology for EEG signal and emotion recognition, applied in medical science, psychological devices, sensors, etc., can solve problems such as poor generalization ability and low accuracy rate
- Summary
- Abstract
- Description
- Claims
- Application Information
AI Technical Summary
Problems solved by technology
Method used
Image
Examples
Embodiment 1
[0091] EEG signal and emotion label preprocessing:
[0092] Select the 8-lead EEG data of the symmetrical left and right brain hemispheres associated with emotions for research. The lead selection is as follows: figure 2 shown. The corresponding leads are Fp1, Fp2, F3, F4, P3, P4, O1, O2. The emotion-induced EEG signal was obtained by subtracting the first 3s data from the last 60s data of the 63s EEG data.
[0093] The two emotional dimensions of valence and arousal are used to classify positive and negative emotions. The present invention selects two emotional label thresholds for valence and arousal to divide emotional states in dimensions. The first type of situation: the emotional label score greater than 7 is regarded as high valence and high arousal, and less than 3 is regarded as low valence and low arousal; the second type of situation: the emotional label score greater than 6 is regarded as high valence and high arousal, and less than 4 is regarded as low Potency...
Embodiment 2
[0097] EEG feature extraction: including frequency domain features, time-frequency features and nonlinear features.
[0098] 1. Frequency domain characteristics
[0099] (1) Map the EEG signal to the four rhythmic frequency bands of θ, α, β, and γ;
[0100] (2) Obtain the power spectrum of the EEG signal by using the AR model method;
[0101] (3) Use the Burg algorithm to quickly realize the power spectrum parameter estimation;
[0102] (4) Calculate the spectral energy corresponding to each rhythm frequency band of the EEG signal as the frequency domain features of the EEG θ, α, β and γ rhythms.
[0103] 2. Time-frequency characteristics
[0104] The EEG signal s(t) is decomposed by the wavelet packet, and the jth layer gets 2 j equal-bandwidth subspaces, subspaces The subsignals of are:
[0105]
[0106] in, for the subspace The wavelet packet decomposition coefficient of , ψ j,k (t) is a wavelet function. The signal s(t) can be refactored as:
[0107]
...
Embodiment 3
[0134] Stacked self-encoded emotion classification and recognition
[0135] 1. Training stacked self-encoder neural network:
[0136] First, the sample data is randomly divided into 5 parts, 4 parts are randomly selected as the training set, and the remaining 1 part is used as the test set.
[0137] The sparse autoencoder is a multi-layer deep neural network composed of an input layer, a hidden layer, and an output layer. The layer-by-layer greedy training method is used to make the output value of the autoencoder infinitely close to the input value. The input layer nodes are EEG feature vectors, the number of output layer nodes is 2, the number of neuron nodes in the hidden layer of the first sparse autoencoder is 15, and the number of neuron nodes in the hidden layer of the second sparse autoencoder is 7.
[0138] The steps to train a stacked autoencoder neural network are:
[0139] Step 1: Input training data n is the number of samples, and m is the feature dimension of...
PUM
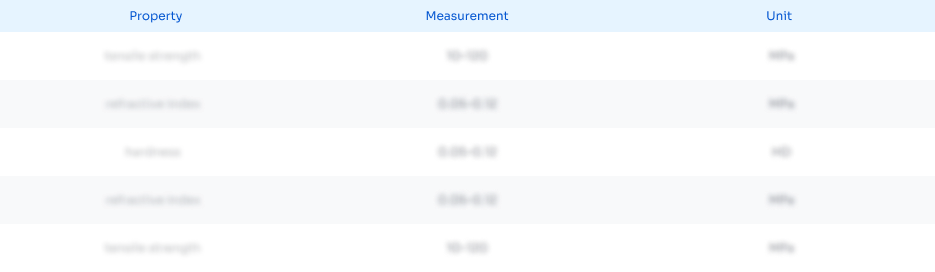
Abstract
Description
Claims
Application Information
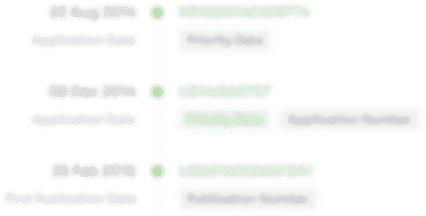
- R&D Engineer
- R&D Manager
- IP Professional
- Industry Leading Data Capabilities
- Powerful AI technology
- Patent DNA Extraction
Browse by: Latest US Patents, China's latest patents, Technical Efficacy Thesaurus, Application Domain, Technology Topic, Popular Technical Reports.
© 2024 PatSnap. All rights reserved.Legal|Privacy policy|Modern Slavery Act Transparency Statement|Sitemap|About US| Contact US: help@patsnap.com