Pulmonary nodule segmentation method for lung CT image
A technology for CT images and pulmonary nodules, applied in the field of medical image processing, can solve problems such as inability to perform overall segmentation, information redundancy, and difficulty in nodule segmentation
Pending Publication Date: 2021-04-30
TONGJI UNIV
View PDF3 Cites 6 Cited by
- Summary
- Abstract
- Description
- Claims
- Application Information
AI Technical Summary
Problems solved by technology
[0003] When the commonly used U-Net and FCN models perform dense prediction, due to the existence of cascaded convolution operations, low-level feature maps are repeatedly extracted, resulting in redundant information, which will cause large errors in medical image detection and segmentation.
And in the shallow layer of the CNN network model, the feature map set has rich spatial information, which is helpful for the prediction of spatial details, but the semantic information is insufficient because the receptive field is too small
At the deep stage of the network, semantic information is sufficient to provide accurate semantic predictions, but lacks spatial details
In addition, the heterogeneity of pulmonary nodules and the presence of similar visual features between nodules and the surrounding environment make the segmentation of nodules difficult
Most importantly, in some CT images, there are too many lung nodules for overall segmentation
Method used
the structure of the environmentally friendly knitted fabric provided by the present invention; figure 2 Flow chart of the yarn wrapping machine for environmentally friendly knitted fabrics and storage devices; image 3 Is the parameter map of the yarn covering machine
View moreImage
Smart Image Click on the blue labels to locate them in the text.
Smart ImageViewing Examples
Examples
Experimental program
Comparison scheme
Effect test
Embodiment
[0058] like figure 1 Shown, a lung nodule segmentation method of lung CT graphics, comprising the following steps:
[0059] S1. Obtain a lung CT image data set, and perform a data enhancement operation on the lung CT image data set;
[0060] S2. Dividing the lung CT image data set after the data enhancement operation into a training set and a test set;
the structure of the environmentally friendly knitted fabric provided by the present invention; figure 2 Flow chart of the yarn wrapping machine for environmentally friendly knitted fabrics and storage devices; image 3 Is the parameter map of the yarn covering machine
Login to View More PUM
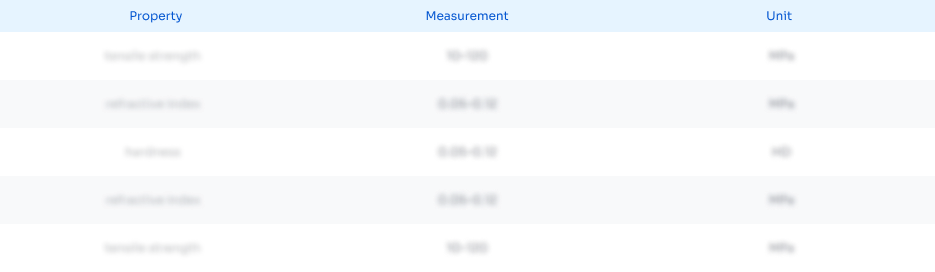
Abstract
The invention relates to a pulmonary nodule segmentation method for a lung CT image, and the method comprises the following steps: obtaining a lung CT image data set, and carrying out the data enhancement of the lung CT image data set; dividing the lung CT image data set after the data enhancement operation into a training set and a test set; constructing a semantic segmentation model based on the enhanced UNet structure, and training and testing the semantic segmentation model by using the training set and the test set to obtain a pulmonary nodule segmentation model; and inputting the actual lung CT image to be measured into the pulmonary nodule segmentation model, and outputting to obtain a corresponding pulmonary nodule segmentation image. Compared with the prior art, the pulmonary nodule segmentation method has the advantages that deep learning and a semantic segmentation technology are combined, an end-to-end semantic segmentation model is provided, and the lung and pulmonary nodule can be automatically, quickly and accurately segmented from the lung CT image by utilizing the ResNet101 backbone network, the context information extraction module and the spatial information extraction module in the semantic segmentation model.
Description
technical field [0001] The invention relates to the technical field of medical image processing, in particular to a method for segmenting pulmonary nodules of CT images of the lungs. Background technique [0002] According to statistics, lung cancer has become one of the deadliest diseases. The number of people who die from lung cancer has exceeded 1.7 million each year, and its mortality rate is rising rapidly year by year. Lung cancer can be detected early by detecting small pulmonary nodules. At present, Convolutional neural networks (CNNs) have played a great role in the detection and analysis of lung CT. In the research on automatic segmentation of medical images, in order to obtain higher segmentation results, many medical image segmentation models use the classic semantic segmentation Architectures, including U-Net, codec architectures, and fully convolutional networks. Shaziya et al. proposed a U-Net ConvNet model for lung CT image segmentation. After the image of ...
Claims
the structure of the environmentally friendly knitted fabric provided by the present invention; figure 2 Flow chart of the yarn wrapping machine for environmentally friendly knitted fabrics and storage devices; image 3 Is the parameter map of the yarn covering machine
Login to View More Application Information
Patent Timeline
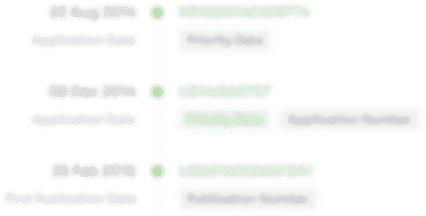
Patent Type & Authority Applications(China)
IPC IPC(8): G06T7/00G06T7/11
CPCG06T7/0012G06T7/11G06T2207/10081G06T2207/20081G06T2207/20084G06T2207/30064
Inventor 韩慧慧王坚
Owner TONGJI UNIV
Who we serve
- R&D Engineer
- R&D Manager
- IP Professional
Why Patsnap Eureka
- Industry Leading Data Capabilities
- Powerful AI technology
- Patent DNA Extraction
Social media
Patsnap Eureka Blog
Learn More Browse by: Latest US Patents, China's latest patents, Technical Efficacy Thesaurus, Application Domain, Technology Topic, Popular Technical Reports.
© 2024 PatSnap. All rights reserved.Legal|Privacy policy|Modern Slavery Act Transparency Statement|Sitemap|About US| Contact US: help@patsnap.com