Unsupervised hyperspectral image change detection method based on adversarial learning
A hyperspectral image and change detection technology, applied in the field of image processing, can solve the problems of the network model's weak ability to distinguish between changing and unchanged test samples, not considering the particularity of hyperspectral image change detection, and low change detection accuracy. The effect of preserving spatial correlation, improving accuracy, and improving detection accuracy
- Summary
- Abstract
- Description
- Claims
- Application Information
AI Technical Summary
Problems solved by technology
Method used
Image
Examples
Embodiment Construction
[0034] The embodiments and effects of the present invention will be further described in detail below in conjunction with the accompanying drawings.
[0035] refer to figure 1 , the implementation steps of this example are as follows:
[0036] Step 1. Construct an unsupervised spectral mapping network Q based on adversarial learning.
[0037] 1.1) Construct a six-layer generation network E based on the spectral angular distance constraints:
[0038] The six-layer generation network E is as follows: input layer → first hidden layer → second hidden layer → spectral feature layer → third hidden layer → fourth hidden layer → output layer, wherein the parameters of each layer are set as follows: The number of nodes in the input layer is set to the total number of bands L of the hyperspectral image, the number of nodes in the first hidden layer and the second hidden layer is 500, the number of nodes in the spectral feature layer is 30, the number of nodes in the third hidden layer...
PUM
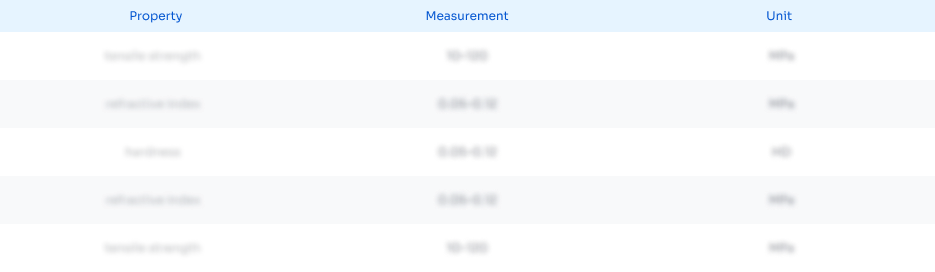
Abstract
Description
Claims
Application Information
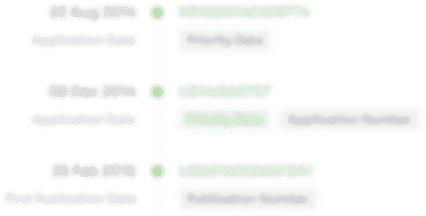
- R&D Engineer
- R&D Manager
- IP Professional
- Industry Leading Data Capabilities
- Powerful AI technology
- Patent DNA Extraction
Browse by: Latest US Patents, China's latest patents, Technical Efficacy Thesaurus, Application Domain, Technology Topic, Popular Technical Reports.
© 2024 PatSnap. All rights reserved.Legal|Privacy policy|Modern Slavery Act Transparency Statement|Sitemap|About US| Contact US: help@patsnap.com