Bidirectional GRU relation extraction data processing method and system, terminal and medium
A technology of relation extraction and data processing, which is applied in the fields of electrical digital data processing, natural language data processing, digital data protection, etc., can solve the problems of model error influence, increase the workload of model calculation, increase the amount of model calculation, etc., and achieve advanced performance , saving computational overhead, avoiding error accumulation and error propagation effects
- Summary
- Abstract
- Description
- Claims
- Application Information
AI Technical Summary
Problems solved by technology
Method used
Image
Examples
Embodiment 1
[0124] The purpose of the present invention is to provide an efficient and accurate deep learning relation extraction method based on keyword attention, which is tested using the SemEval-2010 Task 8 data set, a benchmark data set in the field of relation extraction. First, the method of the present invention processes the data set to obtain a sentence dictionary and an entity relationship dictionary, and calculates the relative position scalar between each word and two entity words, and then converts it into a position feature vector through a position embedding matrix. Next, through the ELMo (embedding from language model) pre-training model, the corpus processed by the NLTK data package is converted into a 512-dimensional word vector, and input into the multi-head attention mechanism, which weights the words with relational expressions in the sentence, and unrelated Words are denoised. Then, the result is input to the Bi-GRU network layer, where the input is context-encoded,...
Embodiment 2
[0181] The relevant experiments of the present invention are based on the TensorFlow environment of Python 3.7, PyCharm 2020.2.2 (Professional Edition), the main data package is TensorFlow 2.5.0-dev20201127 version, cudav11.1, cudnn v8.0.4, pytorch v1.7.
[0182] 1. Data sources and evaluation criteria
[0183] The experiments of the present invention are evaluated on the SemEval-2010 Task 8 dataset, which is a widely used benchmark dataset in the field of relation extraction (see Figure 6 ). The dataset has 19 relationship types, including 9 directional relationships and others: Cause-Effect, Instrument-Agency, Product-Producer, Con-tent-Container, Entity-Origin, Entity-Destination, Component-Whole, ember -Collection, Message-Topic and Other. The data set consists of 10717 sentences, including 8000 training samples and 2717 test samples. The proportion of each label in the training set and test set is shown in Table 1 and Table 2.
[0184] Table 1 Proportion of various da...
PUM
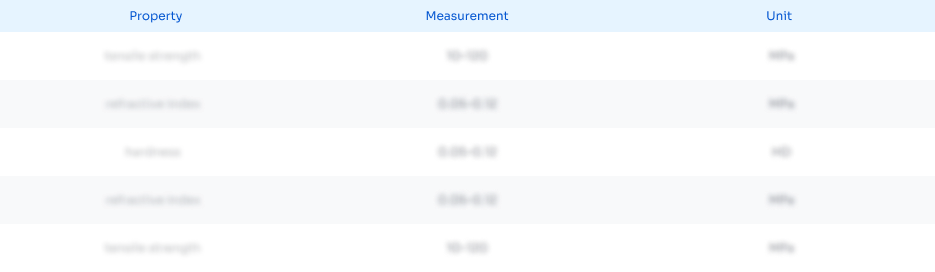
Abstract
Description
Claims
Application Information
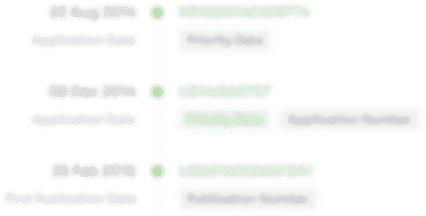
- R&D Engineer
- R&D Manager
- IP Professional
- Industry Leading Data Capabilities
- Powerful AI technology
- Patent DNA Extraction
Browse by: Latest US Patents, China's latest patents, Technical Efficacy Thesaurus, Application Domain, Technology Topic, Popular Technical Reports.
© 2024 PatSnap. All rights reserved.Legal|Privacy policy|Modern Slavery Act Transparency Statement|Sitemap|About US| Contact US: help@patsnap.com