Complex scene 3D point cloud semantic segmentation method based on convolutional neural network
A convolutional neural network and complex scene technology, applied in the field of 3D point cloud semantic segmentation in complex scenes, can solve problems such as fixed, ignoring neighbor point learning, under-segmentation, etc.
- Summary
- Abstract
- Description
- Claims
- Application Information
AI Technical Summary
Problems solved by technology
Method used
Image
Examples
Embodiment Construction
[0032]Embodiments of the present invention will be described in detail below, and examples of the embodiments are illustrated in the drawings, in which the same or similar reference numerals represent the same or similar elements or elements having the same or similar functions. The following is exemplary, and is intended to be used to illustrate the invention without understanding the limitation of the invention.
[0033]In the description of the invention, the meaning of "multiple" is two or more, unless otherwise specifically defined.
[0034]Seefigure 1 The present invention provides a complicated scenario 3D point cloud language segmentation method based on convolutional neural network, including the following steps:
[0035]S101, the obtained original point cloud is sampled, and the sampled sampling point clouds obtained by the center self-focus mechanism and the neighboring self-focus mechanism are separately extracted to obtain the corresponding point cloud spatial position character...
PUM
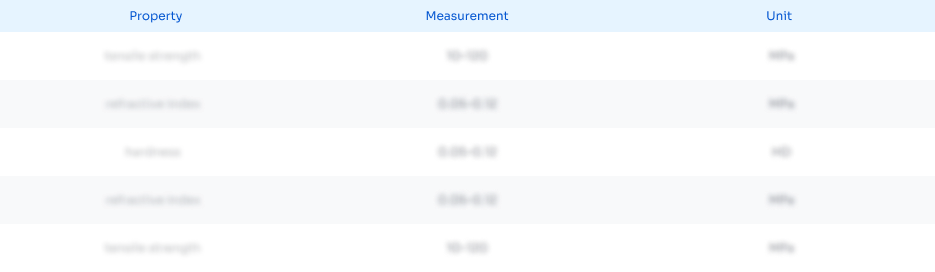
Abstract
Description
Claims
Application Information
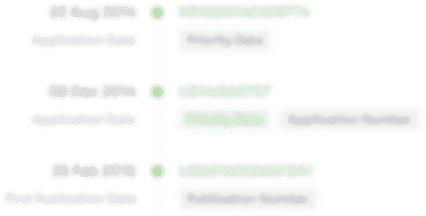
- R&D Engineer
- R&D Manager
- IP Professional
- Industry Leading Data Capabilities
- Powerful AI technology
- Patent DNA Extraction
Browse by: Latest US Patents, China's latest patents, Technical Efficacy Thesaurus, Application Domain, Technology Topic, Popular Technical Reports.
© 2024 PatSnap. All rights reserved.Legal|Privacy policy|Modern Slavery Act Transparency Statement|Sitemap|About US| Contact US: help@patsnap.com