Real image denoising method based on pseudo 3D autocorrelation network
A real image and autocorrelation technology, applied in the fields of computer vision and image processing, can solve the problems of poor denoising effect, high denoising model complexity, and large computational burden, achieving multi-discriminative features, low model complexity, and reduced The effect of computational complexity
- Summary
- Abstract
- Description
- Claims
- Application Information
AI Technical Summary
Problems solved by technology
Method used
Image
Examples
Embodiment Construction
[0024] The present invention will be further described below in conjunction with the accompanying drawings and specific embodiments.
[0025] Embodiments of the present invention propose a real image denoising method based on a pseudo-3D autocorrelation network, which mainly includes steps S1-S4:
[0026] S1. Construct a pseudo 3D auto-correlation block (pseudo 3D auto-correlation blocks, P3AB) based on one-dimensional fast convolution, and the pseudo 3D auto-correlation block is used to perform the following operations:
[0027] First, for the elements at each position of the input feature map, the autocorrelation features in the horizontal direction, vertical direction and channel direction are respectively extracted through one-dimensional fast convolution. After traversing all positions, the horizontal direction, vertical direction and channel direction are respectively obtained. The pseudo 3D autocorrelation features of the direction; secondly, the channel cascade is perf...
PUM
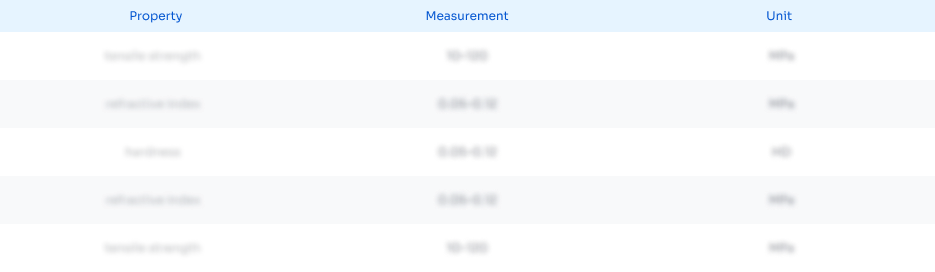
Abstract
Description
Claims
Application Information
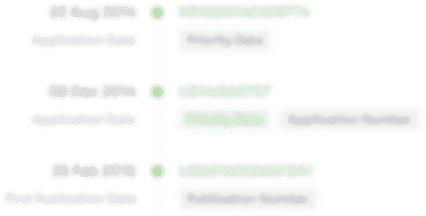
- R&D Engineer
- R&D Manager
- IP Professional
- Industry Leading Data Capabilities
- Powerful AI technology
- Patent DNA Extraction
Browse by: Latest US Patents, China's latest patents, Technical Efficacy Thesaurus, Application Domain, Technology Topic.
© 2024 PatSnap. All rights reserved.Legal|Privacy policy|Modern Slavery Act Transparency Statement|Sitemap