A method for detecting the loss of assembly bolts of a rail vehicle motor-sensing hanger
A rail vehicle and loss detection technology, applied in neural learning methods, neural architecture, image analysis, etc., can solve the problems of false positives and false positives, high cost, low efficiency, etc., to reduce energy and time, achieve good results, and improve training The effect of precision
- Summary
- Abstract
- Description
- Claims
- Application Information
AI Technical Summary
Problems solved by technology
Method used
Image
Examples
specific Embodiment approach 1
[0079] Specific implementation mode one: the following combination Figure 1 to Figure 7 Describe this embodiment, a method for detecting the loss of assembly bolts of a rail vehicle machine-sensing hanger described in this embodiment, the method includes the following steps:
[0080] Step 1. Taking the machine-sensing hanger as the target, continuously collect multiple passing images to obtain continuous frame passing images, and use the SSD detection network to obtain the target area sub-image of the U-shaped groove of the machine-sensing hanger, and then remove the blurred images;
[0081] What is acquired is a set of images, which are multiple images sorted in time series.
[0082] Step 2: Input multiple clear U-groove target area sub-images of the machine-sensing hanger into the fault target segmentation model in time series, and output the predicted image of the U-shaped groove of the machine-sensing hanger used to represent the information of the assembled bolts;
[00...
specific Embodiment approach 2
[0087] Embodiment 2: This embodiment further explains Embodiment 1. The fault target segmentation model includes a convolutional neural network CNN encoder, a convolutional long-short-term memory artificial neural network ConvLSTM and a convolutional neural network CNN decoder. The faulty target segmentation The model building process includes:
[0088] Step 21, establish a training data set; output images in groups to the encoder, each group of images is n consecutive images sorted by time sequence;
[0089] Step 22, using the Resnet network as a reference network to construct a convolutional neural network CNN encoder, the convolutional neural network CNN encoder will generate n feature maps corresponding to n pieces of continuous images input by time series;
[0090] The convolutional neural network CNN encoder inputs consecutive n images X t=1 ,X t=2 ,...,X t=n , output n feature maps Enc t=1 ,Enc t=2 ,...,Enc t=n ;
[0091] Step 2 and 3: Construct a convolutional l...
specific Embodiment approach 3
[0102] Specific implementation mode three: this implementation mode further explains implementation mode two, and the process of establishing a training data set includes the following steps:
[0103] Step A1, collecting a large number of images of passing vehicles with the machine sense hanger as the target;
[0104] Step A2, establishing an original image data set, specifically:
[0105] The SSD detection network is used to train the passing image, the U-shaped groove of the machine-sensing hanger and the area where the machine-sensing hanger is marked, and the sub-map of the target area of the U-shaped groove of the machine-sensing hanger is obtained through the SSD detection network training, and then the machine is established. The original image data set of the U-shaped groove of the sense hanger;
[0106] Step A3, removing blurred images, specifically:
[0107] Edge gradient detection is performed on each U-shaped groove target area sub-image in the original data se...
PUM
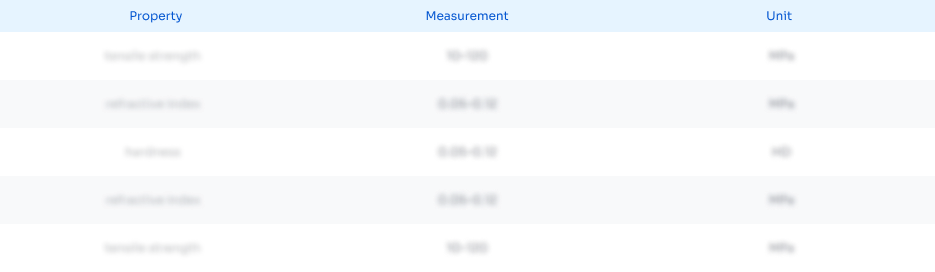
Abstract
Description
Claims
Application Information
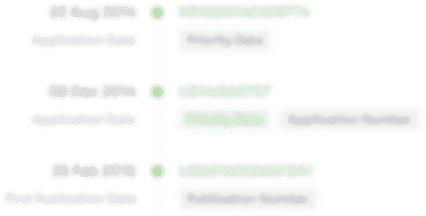
- R&D
- Intellectual Property
- Life Sciences
- Materials
- Tech Scout
- Unparalleled Data Quality
- Higher Quality Content
- 60% Fewer Hallucinations
Browse by: Latest US Patents, China's latest patents, Technical Efficacy Thesaurus, Application Domain, Technology Topic, Popular Technical Reports.
© 2025 PatSnap. All rights reserved.Legal|Privacy policy|Modern Slavery Act Transparency Statement|Sitemap|About US| Contact US: help@patsnap.com