Real-time traffic risk AI prediction method and system based on driving path
A technology of driving path and prediction method, which is applied to the traffic control system of road vehicles, traffic control system, traffic flow detection, etc.
- Summary
- Abstract
- Description
- Claims
- Application Information
AI Technical Summary
Problems solved by technology
Method used
Image
Examples
Embodiment 1
[0067] Such as figure 1 As shown, the real-time traffic risk AI prediction method based on driving path of the present invention includes S1 urban road model establishment of artificial intelligence model to identify urban roads and form a gridded urban road network C , the urban road network C Mesh to form 625 branch networks d 1 , d 2 ,..., d 625(see figure 2 ).
[0068] The artificial intelligence model includes: using the RNN cyclic neural network algorithm to generate road continuous nodes through an encoder and a decoder, and connecting the two nodes before and after generation during the generation process, and inputting new generation nodes into the node generator to continuously generate new nodes, and continue to connect the generated new nodes, so that the loop is connected to form a road network.
[0069] Specifically, the road network extraction process includes: gridding the city, for each grid point g Carry out branch network extraction, each grid poi...
Embodiment 2
[0079] Described step S2 specifically comprises as follows:
[0080] S2-1 Obtain the rush hour for going to work Figure 4 Mid-city road network C a grid of g One of the vehicles in the partial road network with construction points in m i driving route information,
[0081] Wherein, the driving route information includes: currently set driving route, historical route, information of deviation from the route, grid points g The number of vehicles on the same road within the current radius range R=150m is 3, and the number of vehicles on the overlapping road route within the radius range of 150m is 3; no vehicle deviation from the path is found in this embodiment.
[0082] S2-2 The server obtains the currently set driving route planned by the navigation device, then sends the known route information and driving route information to the prediction terminal, and calculates the m i Two of the vehicles on the same road route within a radius of 150m have the currently set trave...
Embodiment 3
[0085] When S2-3 acquires the remaining vehicle whose current driving path is unknown due to the navigation not being turned on n i with the car m i Road alignments have the same road and coincide with historical paths on the same road alignment l k , are also within 150m, and are already at the coincidence, the distance variation is getting smaller and 80m apart. and there is a history path l k is the vehicle's driving path calculated according to the positioning system in the vehicle, and the server records the vehicle's n i Two regular historical routes exist throughout the year l 1 with l 2 , and go from Monday to Friday l 1 while the weekend go l 2 , while traveling the same unconventional driving path only 10 times a year s 1 , then it is considered that the probability of 10 / 365=2 / 73 in a year is to go s 1 And 71 / 73 probability to go l 1 with l 2 . Among them, the probability of 5 / 7×71 / 73 goes l 1 And 2 / 7×71 / 73 probability to go l 2 ,but =5 / ...
PUM
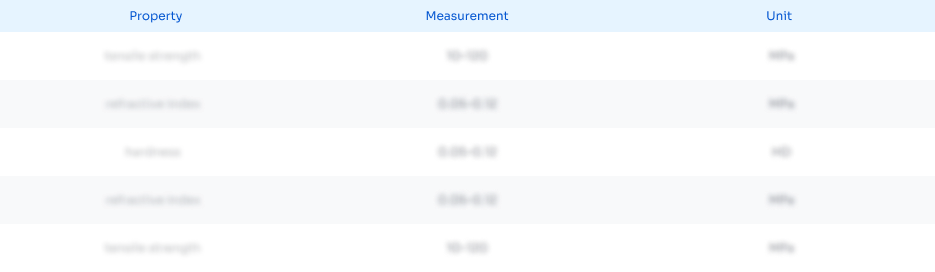
Abstract
Description
Claims
Application Information
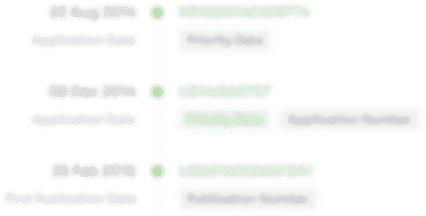
- R&D
- Intellectual Property
- Life Sciences
- Materials
- Tech Scout
- Unparalleled Data Quality
- Higher Quality Content
- 60% Fewer Hallucinations
Browse by: Latest US Patents, China's latest patents, Technical Efficacy Thesaurus, Application Domain, Technology Topic, Popular Technical Reports.
© 2025 PatSnap. All rights reserved.Legal|Privacy policy|Modern Slavery Act Transparency Statement|Sitemap|About US| Contact US: help@patsnap.com