A Generative Adversarial Network for Synthesizing Medical Images
A medical image and network technology, applied in the medical image field of computer vision, can solve problems such as limiting the development of deep learning and scarcity of high-quality data sets, and achieve the effects of alleviating mode collapse, improving training efficiency, and balancing the learning rate.
- Summary
- Abstract
- Description
- Claims
- Application Information
AI Technical Summary
Problems solved by technology
Method used
Image
Examples
specific Embodiment
[0061] Step 1: Download the medical image dataset, and extract a total of 1556 PD images according to the label document. For the images that do not meet the resolution, the cubic interpolation method is used for upsampling, and then 1556 images are saved in npy format.
[0062] Step 2: Construct a progressive network. Initially, only low-resolution image generation can be learned. As the training progresses, the number of layers of the network is continuously deepened, and then higher-resolution image generation is learned. Finally, GANs are continuously updated to enable Learn the generation of 512*512 resolution medical images.
[0063] In the convolution process of the experiment, the use of convolution plus sampling replaces the more commonly used deconvolution in the generated image. The purpose of using convolution plus sampling is to avoid the checkerboard when the size of the convolution kernel is not divisible by the step size. Effect (checkboard), especially when w...
PUM
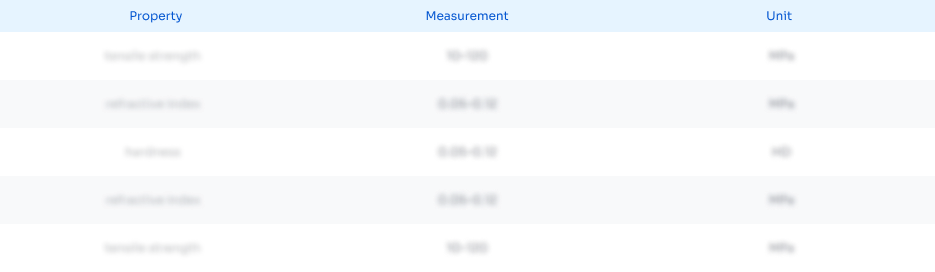
Abstract
Description
Claims
Application Information
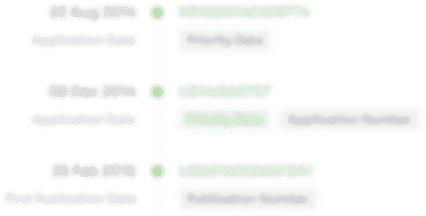
- R&D Engineer
- R&D Manager
- IP Professional
- Industry Leading Data Capabilities
- Powerful AI technology
- Patent DNA Extraction
Browse by: Latest US Patents, China's latest patents, Technical Efficacy Thesaurus, Application Domain, Technology Topic, Popular Technical Reports.
© 2024 PatSnap. All rights reserved.Legal|Privacy policy|Modern Slavery Act Transparency Statement|Sitemap|About US| Contact US: help@patsnap.com