Hyperspectral image classification method and system based on kernel-guided variable convolution and dual-window joint bilateral filter
A bilateral filter and classification method technology, applied in the field of hyperspectral image classification, can solve the problems of salt and pepper noise regional level, misclassification, inability to extract spatial spectrum information, etc., to improve classification accuracy, reduce inter-class similarity and intra-class similarity. Volatility, the effect of improving overall classification performance
- Summary
- Abstract
- Description
- Claims
- Application Information
AI Technical Summary
Problems solved by technology
Method used
Image
Examples
specific Embodiment approach 1
[0049] Aiming at the existing problems of hyperspectral image classification, the present invention proposes a hyperspectral image classification algorithm (KDCDWBF) framework based on kernel-guided variable convolution and dual-window joint filtering, figure 1 As shown, the present invention can combine the advantages of kernel-guided variable convolution and dual-window joint bilateral filter.
[0050] The hyperspectral image classification method based on kernel-guided variable convolution and dual-window joint bilateral filter described in this embodiment includes the following steps:
[0051] The hyperspectral image classification model based on kernel-guided variable convolution and dual-window joint filtering mainly includes two stages of feature extraction and reclassification. The feature extraction stage in the flowchart is the first stage of the algorithm, and the reclassification stage is the second stage of the algorithm.
[0052] 1) In the first stage, the size ...
specific Embodiment approach 2
[0092] The hyperspectral map classification system based on kernel-guided variable convolution and dual-window joint bilateral filter described in this embodiment is used to perform the hyperspectral classification based on kernel-guided variable convolution and dual-window joint bilateral filter Graph classification method.
Embodiment
[0094] Two hyperspectral data sets, PaviaU and SD, are used to illustrate the effect of the hyperspectral image classification algorithm based on kernel-guided variable convolution and dual-window joint filtering proposed by the present invention. The properties of these two datasets are listed in Table 1. The present invention randomly selects 1% and 5% of samples as training and verification data sets, and 94% of samples as test data sets. The experimental results use overall classification accuracy (OA), average classification accuracy (AA) and Kappa coefficient as measurement indicators, and the higher the value of the three indicators, the better the classification result.
[0095] Table 1 Detailed properties of two hyperspectral datasets PaviaU and SD
[0096]
[0097] Although δ s ,δ r Thresholds are parameters that can be adjusted and can affect the final classification results, but it is not necessary to perform parameter analysis on all parameters. Because eve...
PUM
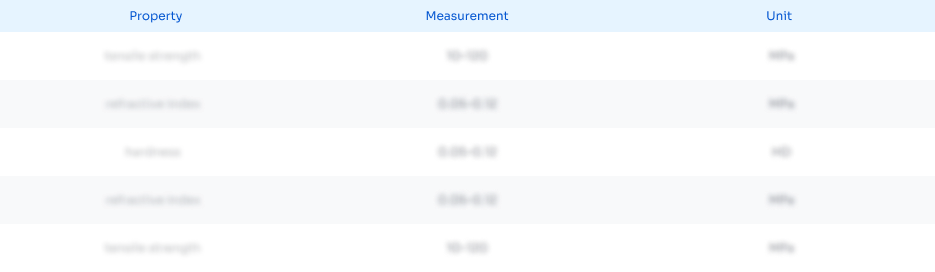
Abstract
Description
Claims
Application Information
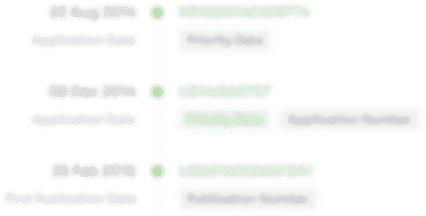
- R&D Engineer
- R&D Manager
- IP Professional
- Industry Leading Data Capabilities
- Powerful AI technology
- Patent DNA Extraction
Browse by: Latest US Patents, China's latest patents, Technical Efficacy Thesaurus, Application Domain, Technology Topic, Popular Technical Reports.
© 2024 PatSnap. All rights reserved.Legal|Privacy policy|Modern Slavery Act Transparency Statement|Sitemap|About US| Contact US: help@patsnap.com