Sample acquisition and rapid labeling method with relatively fixed target state
A target state and sample technology, applied in image analysis, image enhancement, instruments, etc., can solve the problems of difficult sample collection, susceptibility to interference, and restriction of image recognition work
- Summary
- Abstract
- Description
- Claims
- Application Information
AI Technical Summary
Problems solved by technology
Method used
Image
Examples
Embodiment example
[0041] Taking the outdoor AIS switch status recognition scene in a substation in the power industry as an example, assuming that each switch only needs to identify the two states of switch position and close position, the following describes how to obtain a large number of AIS switch samples and quickly mark them. The on-site inspection of the substation includes the number and type of switches, and all the targets to be identified and their different states are divided into several identification categories, the cameras are reasonably selected and the installation locations are arranged, and finally all cameras can be accessed by the picture collection equipment through networking.
[0042] According to the shooting status of the target in the camera, or actually adjust the physical position and angle of the camera, or change the preset position of the camera pan / tilt, and finally realize that all target switches (including the potential opening and closing states of the switch...
PUM
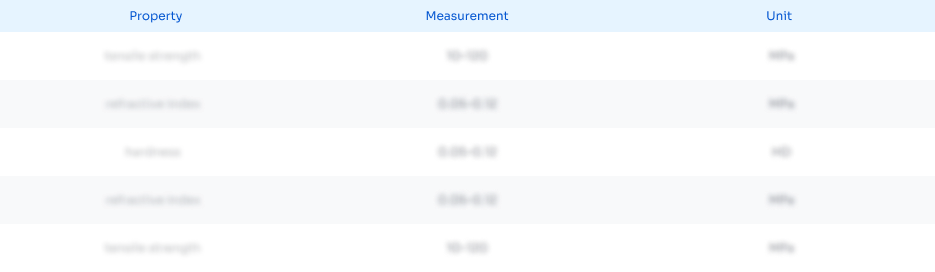
Abstract
Description
Claims
Application Information
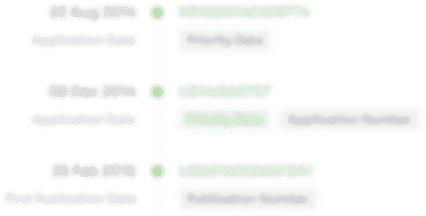
- Generate Ideas
- Intellectual Property
- Life Sciences
- Materials
- Tech Scout
- Unparalleled Data Quality
- Higher Quality Content
- 60% Fewer Hallucinations
Browse by: Latest US Patents, China's latest patents, Technical Efficacy Thesaurus, Application Domain, Technology Topic, Popular Technical Reports.
© 2025 PatSnap. All rights reserved.Legal|Privacy policy|Modern Slavery Act Transparency Statement|Sitemap|About US| Contact US: help@patsnap.com