Neural network design and training method for denoising lightweight real image
A neural network and real image technology, applied in neural learning methods, biological neural network models, image enhancement, etc., can solve problems such as simulation, many neural network parameters, and unfavorable neural network training
- Summary
- Abstract
- Description
- Claims
- Application Information
AI Technical Summary
Problems solved by technology
Method used
Image
Examples
Embodiment Construction
[0038] Below in conjunction with accompanying drawing, the present invention is described in further detail:
[0039] 1. The present invention designs a denoising network combined with a second-order residual attention module
[0040] The present invention designs a novel real image denoising network structure combined with a second-order residual attention module. The denoising network adopts a 4-scale U-shaped network structure, and the overall structure of the network is as follows: figure 1 shown.
[0041] The denoising network consists of 7 second-order residual blocks (2ndRB) and 3 wavelet downsampling layers and 3 wavelet upsampling layers in total. Name 2ndRB as 2ndRB according to the distance from the input 1 ...2nd RB 7 . For the input noisy image, first go through 2ndRB 1 Extract features, 2ndRB 1 The output of has two branches: the first branch is passed to the back of the network, and the 2ndRB 6 The outputs are summed and input to 2ndRB 7 Middle; the sec...
PUM
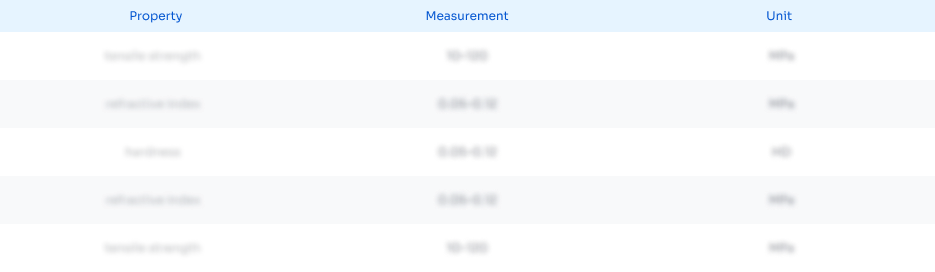
Abstract
Description
Claims
Application Information
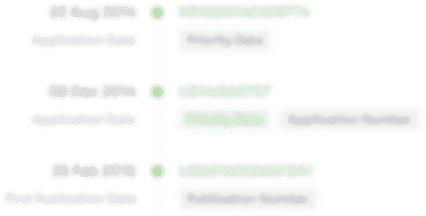
- R&D
- Intellectual Property
- Life Sciences
- Materials
- Tech Scout
- Unparalleled Data Quality
- Higher Quality Content
- 60% Fewer Hallucinations
Browse by: Latest US Patents, China's latest patents, Technical Efficacy Thesaurus, Application Domain, Technology Topic, Popular Technical Reports.
© 2025 PatSnap. All rights reserved.Legal|Privacy policy|Modern Slavery Act Transparency Statement|Sitemap|About US| Contact US: help@patsnap.com