Mechanical fault feature extraction method based on wavelet transform and topological data analysis
A wavelet transform and topology data technology, applied in the field of feature extraction, can solve the problems of learning the nonlinear relationship, complexity and instability of fault data, and achieve the effect of improving the classification accuracy.
- Summary
- Abstract
- Description
- Claims
- Application Information
AI Technical Summary
Problems solved by technology
Method used
Image
Examples
Embodiment 1
[0052] A mechanical fault feature extraction method based on wavelet transform and topological data analysis, comprising the following steps:
[0053] 1. Select the original mechanical vibration data as the training set, perform wavelet transformation on each sample in the training set, and convert the time-domain diagram of the original mechanical vibration signal into a corresponding time-frequency diagram;
[0054] Wavelet transform is a technique aimed at the non-stationary characteristics of signals, which can well reflect the correlation characteristics of signals. Wavelet transform is a time-frequency analysis method with a fixed window size, but the shape of the window can be changed to make it multi-resolution. Wavelet transform has the ability of local analysis in time domain and frequency domain, and is widely used in the diagnosis of mechanical parts.
[0055] The formula of wavelet transform is as follows:
[0056]
[0057] Among them, α is the scale to contr...
experiment example 1
[0082] Taking the bearing fault vibration data set of Case Western Reserve University as an example, the method is described in detail.
[0083] Case Western Reserve data set contains vibration data under four different working conditions (0HP, 1HP, 2HP, 3HP), and also contains data of one normal state and three fault states (inner ring fault, outer ring fault, Rolling element faults), each fault is divided into different fault diameters, so there are nine fault types in the entire data set. In this embodiment, experiments are carried out on data sets with the same fault size and different loads. This solution can also use data of different fault sizes or different loads, this example is for reference only.
[0084] Step 1: Perform preprocessing on the original vibration training data. The preprocessing refers to segmenting the original vibration data set. Every 1024 data forms a sample data. Firstly, wavelet transform is performed on the original vibration data. Since there ...
PUM
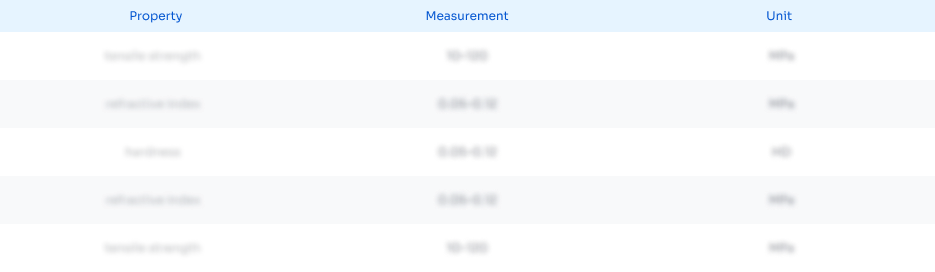
Abstract
Description
Claims
Application Information
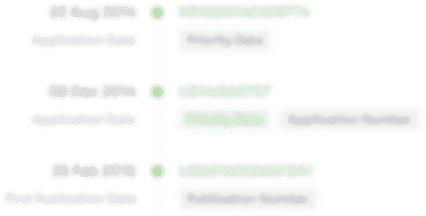
- R&D Engineer
- R&D Manager
- IP Professional
- Industry Leading Data Capabilities
- Powerful AI technology
- Patent DNA Extraction
Browse by: Latest US Patents, China's latest patents, Technical Efficacy Thesaurus, Application Domain, Technology Topic, Popular Technical Reports.
© 2024 PatSnap. All rights reserved.Legal|Privacy policy|Modern Slavery Act Transparency Statement|Sitemap|About US| Contact US: help@patsnap.com