Mixed-experience multi-agent reinforcement learning motion planning method
A multi-agent, motion planning technology, applied in the field of deep learning, can solve problems such as difficulty in adapting to dynamic and complex environments, poor training stability, not caring about the environment, etc., to achieve the effect of accelerating training speed, improving training skills, and reducing update frequency
- Summary
- Abstract
- Description
- Claims
- Application Information
AI Technical Summary
Problems solved by technology
Method used
Image
Examples
specific Embodiment
[0178] 1. Establish a stochastic game model for multi-agent motion planning in complex environments.
[0179] This embodiment belongs to the problem of multi-agent reinforcement learning, and uses stochastic countermeasures as the environment model.
[0180] 1.1. Set the physical model of the agent and the obstacle. The schematic diagram of the model is as follows figure 2 shown.
[0181] The intelligent body is set as a round smart car, and the number is n, and n=5 is set in this embodiment. In the present invention, it is assumed that the physical models of all agents are the same, and for agent i, its radius is set to r i a =0.5m, the velocity is u i =1.0m / s, velocity angle ψ i Indicates the angle between the speed and the positive direction of the X-axis, and the range is (-π, π]. The target of agent i is set to the radius r i g = 1.0m circular area, the location is The distance from agent i is D(P i a ,P i g ). When D(P i a ,P i g )≤r i a + r i g , ...
PUM
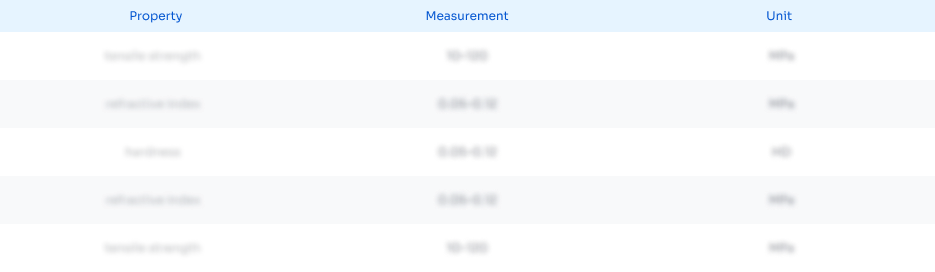
Abstract
Description
Claims
Application Information
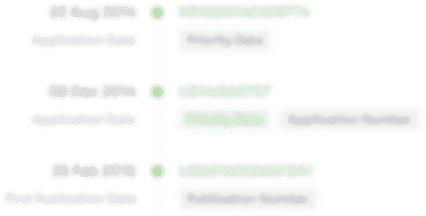
- R&D
- Intellectual Property
- Life Sciences
- Materials
- Tech Scout
- Unparalleled Data Quality
- Higher Quality Content
- 60% Fewer Hallucinations
Browse by: Latest US Patents, China's latest patents, Technical Efficacy Thesaurus, Application Domain, Technology Topic, Popular Technical Reports.
© 2025 PatSnap. All rights reserved.Legal|Privacy policy|Modern Slavery Act Transparency Statement|Sitemap|About US| Contact US: help@patsnap.com