Product key part life end-to-end prediction method based on self-attention network
A prediction method and attention technology, applied in prediction, neural learning methods, biological neural network models, etc., can solve the problems of reducing the prediction accuracy of the model, complicating the implementation process, increasing the difficulty of applying existing prediction methods, etc., and achieving strong time series features The effect of extraction ability
- Summary
- Abstract
- Description
- Claims
- Application Information
AI Technical Summary
Problems solved by technology
Method used
Image
Examples
specific Embodiment
[0034]In this embodiment, the bearing monitoring data of the 2012 PHM data competition (PHM2012 data set for short) is used to carry out experimental verification on a self-attention network-based end-to-end life prediction method for key product parts proposed by the present invention.
[0035] The PHM2012 data set is obtained by performing accelerated degradation experiments on rolling bearings on the PRONOSTIA platform (degradation experiment platform), which mainly includes a rotating part, a loading part and a measuring part. The rotating part is driven by a motor, so that the rolling bearing is in an uninterrupted working state; in order to accelerate the degradation of the bearing, the loading part applies a controllable radial load to the running rolling bearing; the measuring part uses two mutually perpendicular acceleration sensors to measure the rolling bearing level and Vibration signals in two vertical directions. Among them, the acceleration sensor samples once e...
PUM
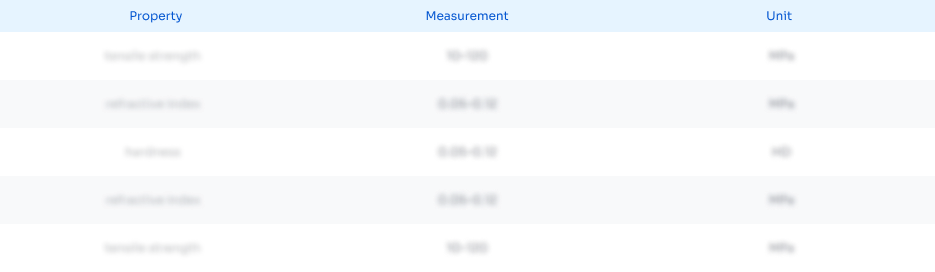
Abstract
Description
Claims
Application Information
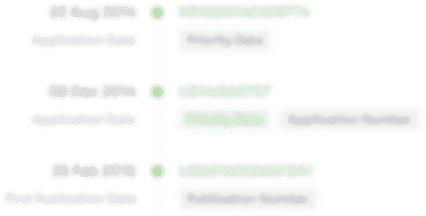
- R&D Engineer
- R&D Manager
- IP Professional
- Industry Leading Data Capabilities
- Powerful AI technology
- Patent DNA Extraction
Browse by: Latest US Patents, China's latest patents, Technical Efficacy Thesaurus, Application Domain, Technology Topic.
© 2024 PatSnap. All rights reserved.Legal|Privacy policy|Modern Slavery Act Transparency Statement|Sitemap