A Method for Predicting the Future Speed Trajectory of a Hybrid Electric Bus
A technology of hybrid power and trajectory prediction, applied in neural learning methods, traffic flow detection, traffic control systems of road vehicles, etc., can solve problems such as insufficient prediction accuracy of future driving conditions
- Summary
- Abstract
- Description
- Claims
- Application Information
AI Technical Summary
Problems solved by technology
Method used
Image
Examples
Embodiment Construction
[0039] The specific embodiments of the present invention will be described in detail below in conjunction with the technical solutions and accompanying drawings.
[0040] Taking the hybrid city bus actually running in a certain place as the research object, such as figure 1 Shown is the schematic diagram of the future vehicle speed trajectory prediction method based on RBF neural network online learning. The core is RBF neural network construction and offline training, and RBF neural network online prediction of future vehicle speed trajectory. It includes the following steps:
[0041] A. Acquisition and normalization of parameters
[0042] A1. Acquisition of parameters: Based on the on-board information acquisition system, the real-time operation data of each data point is collected by different drivers when driving on different road conditions, and stored in the road database. For example, randomly select 4 drivers and 5 driving routes of hybrid electric buses, record the ...
PUM
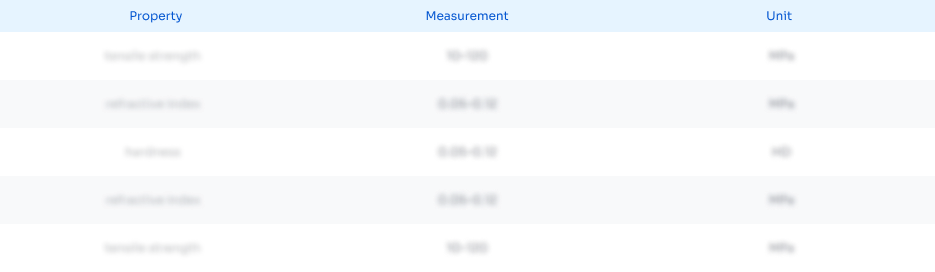
Abstract
Description
Claims
Application Information
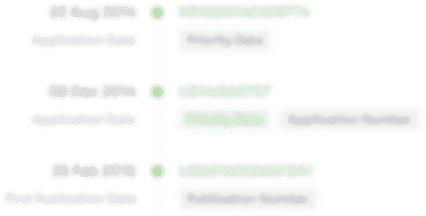
- R&D Engineer
- R&D Manager
- IP Professional
- Industry Leading Data Capabilities
- Powerful AI technology
- Patent DNA Extraction
Browse by: Latest US Patents, China's latest patents, Technical Efficacy Thesaurus, Application Domain, Technology Topic, Popular Technical Reports.
© 2024 PatSnap. All rights reserved.Legal|Privacy policy|Modern Slavery Act Transparency Statement|Sitemap|About US| Contact US: help@patsnap.com