Integrated transfer learning classification method and system for single-view full-polarization SAR data
A technology of transfer learning and classification method, which is applied in the field of full-polarization radar remote sensing image processing, can solve the problems that classification results are easily affected by speckle noise, reduce spatial resolution, and lose spatial details, so as to reduce overfitting and negative Migration risk, improve migration robustness, reduce the effect of high dependency
- Summary
- Abstract
- Description
- Claims
- Application Information
AI Technical Summary
Problems solved by technology
Method used
Image
Examples
Embodiment Construction
[0039] The technical solution of the present invention will be described in detail below in conjunction with the drawings and embodiments.
[0040] In general, POLSAR original single-view data contains 4 channel data acquired under a specific polarization base (generally horizontal-vertical polarization base), so each pixel corresponds to a 2×2 complex matrix, called S 2 Scattering matrix:
[0041]
[0042] When the premise of reciprocity is satisfied, the matrix can be vectorized into a 3-dimensional complex scattering vector Ω as follows without loss of generality:
[0043] where the matrix elements s XX , s XY , s YX , s YY Respectively represent the combination of transmitting X polarized waves-receiving X polarized waves, transmitting Y polarized waves-receiving X polarized waves, transmitting X polarized waves-receiving Y polarized waves, transmitting Y polarized waves-receiving Y polarized waves The complex backscatter coefficient under . X and Y are general...
PUM
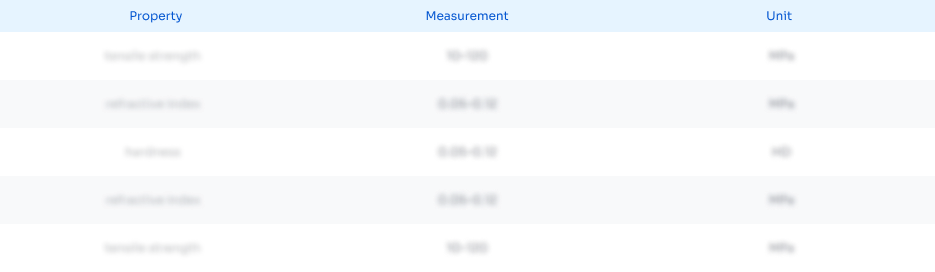
Abstract
Description
Claims
Application Information
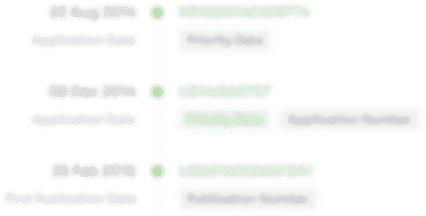
- R&D Engineer
- R&D Manager
- IP Professional
- Industry Leading Data Capabilities
- Powerful AI technology
- Patent DNA Extraction
Browse by: Latest US Patents, China's latest patents, Technical Efficacy Thesaurus, Application Domain, Technology Topic, Popular Technical Reports.
© 2024 PatSnap. All rights reserved.Legal|Privacy policy|Modern Slavery Act Transparency Statement|Sitemap|About US| Contact US: help@patsnap.com