A vehicle trajectory prediction and driving behavior analysis method
A vehicle trajectory and behavior analysis technology, applied in the direction of registration/instruction of vehicle operation, registration/instruction, instrument, etc., can solve problems such as sparse adjacency matrix, avoid underfitting and overfitting, improve the overall prediction effect, The effect of guaranteeing efficiency and precision
- Summary
- Abstract
- Description
- Claims
- Application Information
AI Technical Summary
Problems solved by technology
Method used
Image
Examples
Embodiment Construction
[0057] Attached below Figures 1 to 4 The preferred embodiment of the present invention is described further:
[0058] Step1: Model input data processing
[0059] Before the model is trained, the data needs to be processed into the required form. The trajectory data input for trajectory prediction is processed by a semi-global graph algorithm to generate an adjacency matrix and a feature matrix as the input of the model. Introduce respectively below.
[0060] (1) Trajectory data:
[0061] The original trajectory data is a two-dimensional table, each row is a data sampling point, including information such as time stamp, vehicle identification number, local coordinates, etc., sliced according to time (the length of each segment is the history step plus the prediction step). Among them, the historical vehicle trajectory of the driving scene consists of vehicle trajectory data of τ historical time steps: The trajectory data at each time step is It consists of the local ...
PUM
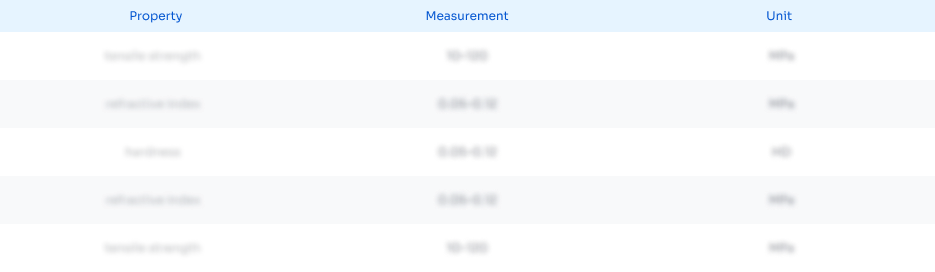
Abstract
Description
Claims
Application Information
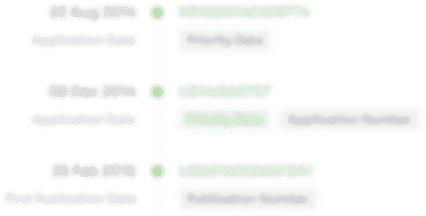
- R&D
- Intellectual Property
- Life Sciences
- Materials
- Tech Scout
- Unparalleled Data Quality
- Higher Quality Content
- 60% Fewer Hallucinations
Browse by: Latest US Patents, China's latest patents, Technical Efficacy Thesaurus, Application Domain, Technology Topic, Popular Technical Reports.
© 2025 PatSnap. All rights reserved.Legal|Privacy policy|Modern Slavery Act Transparency Statement|Sitemap|About US| Contact US: help@patsnap.com