Intrusion detection method based on active learning
A technology of active learning and intrusion detection, applied to instruments, character and pattern recognition, computer components, etc., can solve problems such as poor attack detection effect and poor detection effect of small samples
- Summary
- Abstract
- Description
- Claims
- Application Information
AI Technical Summary
Problems solved by technology
Method used
Image
Examples
Example Embodiment
[0044] Example 1
[0045] refer to Figure 1 ~ Figure 3 , which is the first embodiment of the present invention, provides an active learning-based intrusion detection method, specifically including:
[0046] S1: Use system logs to collect historical data and perform preprocessing to obtain a label sample data set.
[0047] S2: Build a detection and classification model based on the active learning strategy, and combine the semi-supervised transductive support vector machine to train the detection and classification model to form a detection multi-classifier.
[0048] S3: Use the K-Means clustering algorithm for cluster analysis, and combine the trained detection and classification models to output the detection results.
[0049] Preprocessing includes: normalization processing.
[0050] Active learning strategies include: membership query, flow-based selective sampling, and pool-based selective sampling.
[0051] refer to figure 2 , the membership query includes that th...
Example Embodiment
[0088] Example 2
[0089] This embodiment is different from the first embodiment in that it provides an active learning-based intrusion detection method to solve the detailed verification of labeled samples and iterative training, including:
[0090] (1) Select the required labeled samples.
[0091]For example: the labeling of paired samples in TSVM, this method has the advantage of high labeling accuracy, but the labeling speed is very low; the region-based labeling method can label multiple samples at a time, this method has fast labeling speed, but the labeling accuracy is not good It can be guaranteed; this embodiment proposes a method based on multi-classifier voting decision labeling, and selects m samples belonging to more than half of the classifiers in the boundary area at the same time (that is, the minority obeys the majority) for labeling. If the m samples satisfy the maximum classification super plane, it is labeled as a positive class; if m samples satisfy the m...
PUM
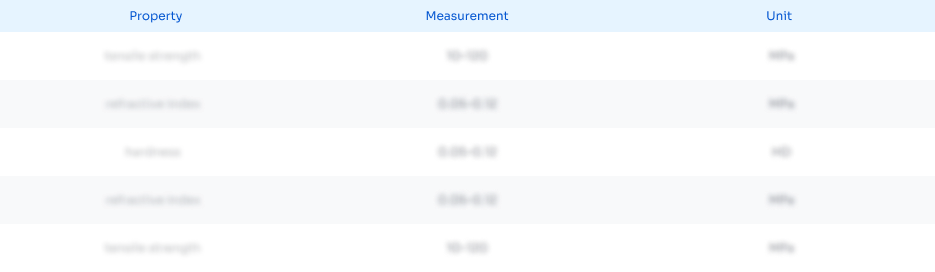
Abstract
Description
Claims
Application Information
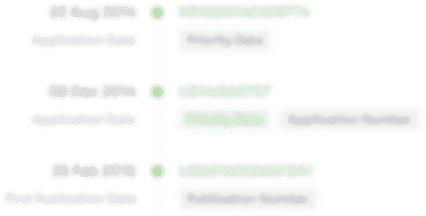
- R&D Engineer
- R&D Manager
- IP Professional
- Industry Leading Data Capabilities
- Powerful AI technology
- Patent DNA Extraction
Browse by: Latest US Patents, China's latest patents, Technical Efficacy Thesaurus, Application Domain, Technology Topic.
© 2024 PatSnap. All rights reserved.Legal|Privacy policy|Modern Slavery Act Transparency Statement|Sitemap