Near infrared spectrum classification model training method and system and classification method and system
A technology of near-infrared spectroscopy and training methods, applied in biological neural network models, character and pattern recognition, instruments, etc., can solve problems such as weak useful information intensity, high noise interference, and inability to ensure the accuracy of classification results
- Summary
- Abstract
- Description
- Claims
- Application Information
AI Technical Summary
Problems solved by technology
Method used
Image
Examples
Embodiment 1
[0045] This embodiment is used to provide a classification model training method for near-infrared spectroscopy, such as figure 1 As shown, the training method includes:
[0046] S1: Obtain multiple near-infrared spectrum sequences for training corresponding to various types of samples;
[0047] Each type of sample corresponds to a preset wavelength range, and the near-infrared spectrum sequence of the sample in the preset wavelength range can be expressed as X={x 1 , x 2 ,...,x i ,...,x n}, n is the number of sequence points in the near-infrared spectrum sequence, and is also the total number of characteristic wavelengths; x i is the absorbance value corresponding to the sample when the wavelength is i.
[0048] For each type of sample, multiple near-infrared spectrum sequences are obtained as training near-infrared spectrum sequences, and the number of training near-infrared spectrum sequences corresponding to different types of samples can be the same or different. Th...
Embodiment 2
[0103] This embodiment is used to provide a kind of near-infrared spectrum classification model training system, such as Figure 12 As shown, the training system includes:
[0104] The first acquisition module M1 is used to acquire multiple training near-infrared spectrum sequences corresponding to various types of samples;
[0105] The first conversion module M2 is used to convert each of the training near-infrared spectral sequences into training two-dimensional images using MTF to obtain training samples; all the training samples and the labels corresponding to the training samples form a training data set ;
[0106] A building block M3 for building an initial classification model;
[0107] The training module M4 is configured to use the training data set to train the initial classification model to obtain a classification model.
[0108] A near-infrared spectrum classification model training system based on Markov transition field (MTF) image coding and residual network...
Embodiment 3
[0110] This embodiment is used to provide a classification method of near-infrared spectrum, such as Figure 13 As shown, the method includes:
[0111] T1: Obtain the near-infrared spectrum sequence to be classified;
[0112] The form of the obtained near-infrared spectrum sequence to be classified is the same as that in S1 in Example 1.
[0113] T2: Using MTF to convert the near-infrared spectrum sequence to be classified into a two-dimensional image;
[0114] As an optional implementation, the near-infrared spectrum sequence to be classified can be clipped and normalized first, which is the same as the method described in Example 1, and the method of converting into a two-dimensional image is also the same as the method used in Example 1 , after being converted into a two-dimensional image, the two-dimensional image can also be further cropped, and the cropping method used is the same as that used in Embodiment 1, and will not be repeated here.
[0115] T3: Using the two-di...
PUM
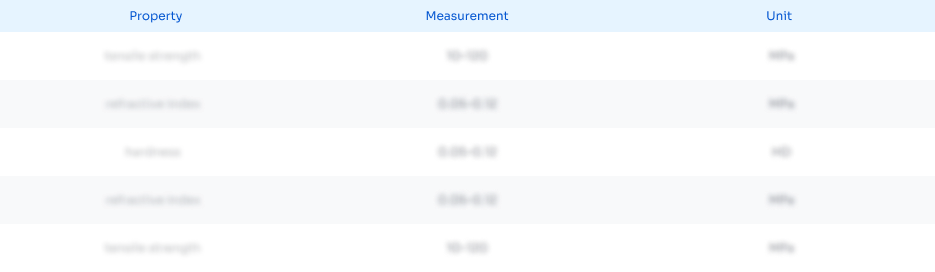
Abstract
Description
Claims
Application Information
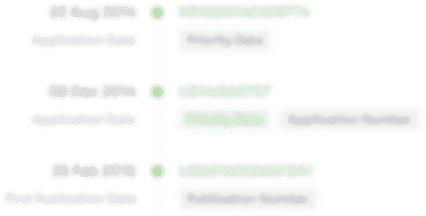
- R&D Engineer
- R&D Manager
- IP Professional
- Industry Leading Data Capabilities
- Powerful AI technology
- Patent DNA Extraction
Browse by: Latest US Patents, China's latest patents, Technical Efficacy Thesaurus, Application Domain, Technology Topic, Popular Technical Reports.
© 2024 PatSnap. All rights reserved.Legal|Privacy policy|Modern Slavery Act Transparency Statement|Sitemap|About US| Contact US: help@patsnap.com