Construction waste remote sensing image recognition method based on deep learning
A construction waste, remote sensing image technology, applied in neural learning methods, character and pattern recognition, instruments, etc., can solve the problems of inability to mine overall information, low recognition accuracy, and remote sensing image recognition methods are easily interfered, and achieve dynamic tracking. Monitor the effect of purifying the urban environment, shortening the revisit cycle, and saving manpower
- Summary
- Abstract
- Description
- Claims
- Application Information
AI Technical Summary
Problems solved by technology
Method used
Image
Examples
specific Embodiment approach 1
[0026] A deep learning-based remote sensing image recognition method for construction waste in this embodiment, such as figure 1 As shown, the method is realized through the following steps:
[0027] Step 1. Preprocessing the acquired remote sensing image to obtain a remote sensing image dataset;
[0028] Step 2, expand the sample of the remote sensing image data set, add L2 regularization penalty term in the seventh layer of neural network, use the expanded data set to train the network model adding L2 regularization penalty term, and obtain the target recognition model;
[0029] Step 3. Improve the semantic segmentation algorithm by calculating the mIOU ratio of the intersection and union of the real value and the predicted value in DeepLab's semantic segmentation method;
[0030] Step 4, using the improved recognition model and algorithm for image recognition.
specific Embodiment approach 2
[0031] The difference from Embodiment 1 is that in this embodiment, a deep learning-based remote sensing image recognition method for construction waste, the step of preprocessing the acquired remote sensing image described in Step 1 to obtain a remote sensing image dataset includes:
[0032] Due to the influence of the overall positioning accuracy of the remote sensing information platform and the sensor error rate, the layers of the panchromatic and multispectral images in the satellite remote sensing technology image may not be aligned, etc., requiring more preprocessing operations on the satellite remote sensing image, using the ENVI platform Perform remote sensing image preprocessing operations such as orthorectification and image fusion on remote sensing images, and perform histogram averaging operations on the resulting data; improve the relative position accuracy of remote sensing data, improve image quality, and enhance data features.
specific Embodiment approach 3
[0033] The difference from the specific embodiment 1 or 2 is that, in this embodiment, a construction waste remote sensing image recognition method based on deep learning, the step of expanding the sample of the remote sensing image dataset described in step 2 adopts an improved generative confrontation network method The features of multiple images are fused, and the specific steps include:
[0034] Aiming at the problem of fewer samples in the building remote sensing data set, the sample expansion experiment of image generation is carried out, and urban construction waste is detected from the perspective of semantic segmentation of remote sensing images, so as to provide a reliable data source expansion method for remote sensing monitoring of urban construction waste, and provide a basis for the management of construction waste stockpiles. provide technical support. Improve the generative confrontation network, improve the accuracy of the semantic segmentation network, and m...
PUM
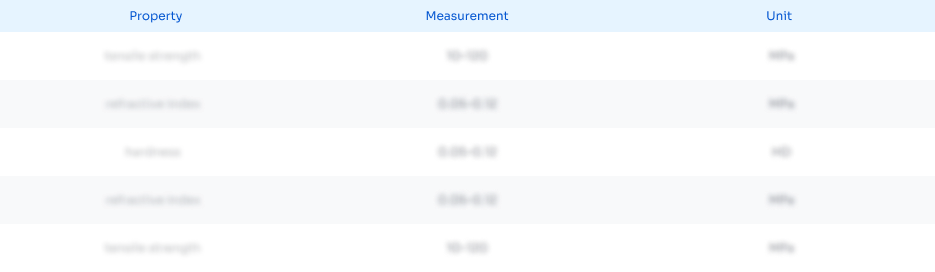
Abstract
Description
Claims
Application Information
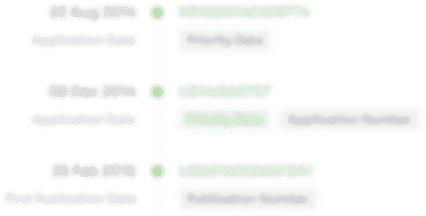
- R&D Engineer
- R&D Manager
- IP Professional
- Industry Leading Data Capabilities
- Powerful AI technology
- Patent DNA Extraction
Browse by: Latest US Patents, China's latest patents, Technical Efficacy Thesaurus, Application Domain, Technology Topic, Popular Technical Reports.
© 2024 PatSnap. All rights reserved.Legal|Privacy policy|Modern Slavery Act Transparency Statement|Sitemap|About US| Contact US: help@patsnap.com