Recommendation method of spatial adaptive graph convolutional network
A technology of convolutional network and recommendation method, applied to the space-adaptive graph convolutional network model in the field of item recommendation, which can solve the problems of unconsidered, highly sensitive negative sampler quality, no further capture of cooperative similarity propagation, etc., to reduce Time complexity, the effect of improving training efficiency
- Summary
- Abstract
- Description
- Claims
- Application Information
AI Technical Summary
Problems solved by technology
Method used
Image
Examples
example
[0050] For user u and item i, a single feature representation is obtained by:
[0051]
[0052] In the Tensorflow environment of actual model training, the example of the present invention uses efficient sparse matrix operations for encoding calculations, and the bilinear graph convolution module is implemented in the form of a matrix as:
[0053] in is the user-item interaction matrix, the element r in this matrix ui = 1 means that user u interacts with item i, otherwise r ui =0. Then, the user-item interaction network The adjacency matrix for is:
[0054]
[0055] make Represents the 0-layer embedding matrix of the input graph convolution module, where The matrix form of user-item co-similarity influence propagation:
[0056]
[0057] in, is the adjacency matrix after symmetric normalization, Indicates that the adjacency matrix A is regularized, and D indicates that degree matrix.
[0058] Finally, the embedding matrix E of users and items in the...
PUM
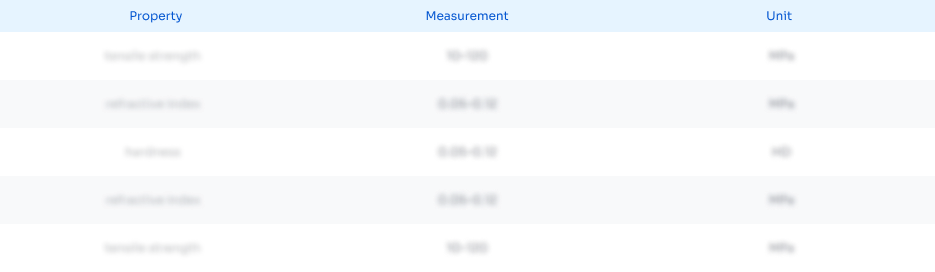
Abstract
Description
Claims
Application Information
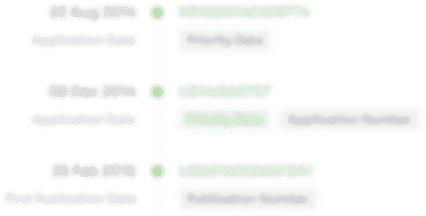
- R&D
- Intellectual Property
- Life Sciences
- Materials
- Tech Scout
- Unparalleled Data Quality
- Higher Quality Content
- 60% Fewer Hallucinations
Browse by: Latest US Patents, China's latest patents, Technical Efficacy Thesaurus, Application Domain, Technology Topic, Popular Technical Reports.
© 2025 PatSnap. All rights reserved.Legal|Privacy policy|Modern Slavery Act Transparency Statement|Sitemap|About US| Contact US: help@patsnap.com