Cross-scene human body action recognition method based on adversarial meta-learning
A human action recognition and human action technology, applied in the fields of wireless network, human action recognition and deep learning, can solve the problems of large fluctuation of CSI action signal, large repetitive workload of action recognition, large manpower and material cost, etc. Classification effect, improve identification ability, improve the effect of accuracy
- Summary
- Abstract
- Description
- Claims
- Application Information
AI Technical Summary
Problems solved by technology
Method used
Image
Examples
Embodiment Construction
[0042] In this embodiment, refer to figure 1 , a cross-scene human action recognition method based on adversarial meta-learning is carried out as follows:
[0043] Step 1. Select two rooms with different indoor environment layouts as scene 1 and scene 2, and deploy a pair of WIFI transceivers respectively:
[0044] The WIFI signal sending device in the WIFI transceiver device is a router with a root antenna, denoted as AP, and the receiving device of WIFI signal is a wireless network card with b antennas, denoted as RP, and the distance between the router AP and the wireless network card RP The distance is l, so that in scenario 1 and scenario 2, a×b antenna pairs are respectively formed for sending and receiving radio signals, and each antenna pair has z available subcarriers;
[0045] In this example, if figure 2 , image 3 As shown, WIFI devices are deployed in fixed positions at intervals of 2.6 meters in the rooms in Scenario 1 and Scenario 2: TL-WDR6500 router with 2...
PUM
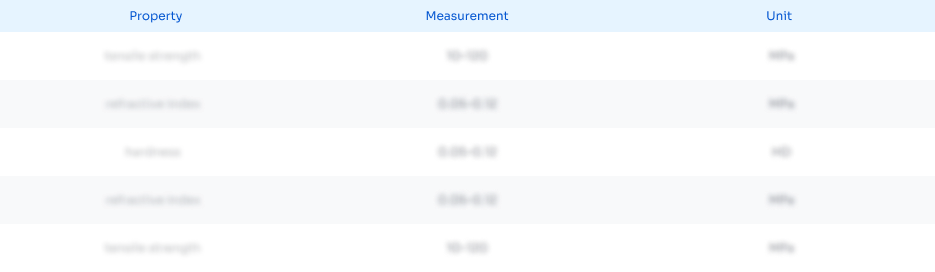
Abstract
Description
Claims
Application Information
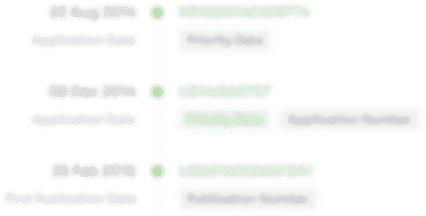
- R&D
- Intellectual Property
- Life Sciences
- Materials
- Tech Scout
- Unparalleled Data Quality
- Higher Quality Content
- 60% Fewer Hallucinations
Browse by: Latest US Patents, China's latest patents, Technical Efficacy Thesaurus, Application Domain, Technology Topic, Popular Technical Reports.
© 2025 PatSnap. All rights reserved.Legal|Privacy policy|Modern Slavery Act Transparency Statement|Sitemap|About US| Contact US: help@patsnap.com