Full-type aortic dissection true and false cavity image segmentation method and system
A technology for aortic dissection and image segmentation, which is applied in image analysis, image data processing, neural learning methods, etc., can solve the problems of low segmentation accuracy and non-automatic segmentation process, and achieve the effect of improving accuracy
- Summary
- Abstract
- Description
- Claims
- Application Information
AI Technical Summary
Problems solved by technology
Method used
Image
Examples
Embodiment 1
[0059] like figure 1 As shown, an embodiment of the present invention provides a method for segmenting true and false lumen images of all types of aortic dissection, the method comprising:
[0060] S1. Obtain images of true and false cavities to be segmented;
[0061] After obtaining the true and false cavity images to be segmented, the preprocessing process should be performed first, including:
[0062] (1) Data dimension normalization
[0063] On the one hand, the input of the network model requires the same data dimension, and on the other hand, big data training has a greater demand for computer video memory. Therefore, in the first step, we need to normalize the data dimension and use the nearest neighbor interpolation algorithm. The zoom function in the ndimage library can achieve this function. Next, to reduce the influence of other tissue areas, crop the central area containing the aorta.
[0064] (2) Numerical normalization and grayscale transformation
[0065] S...
PUM
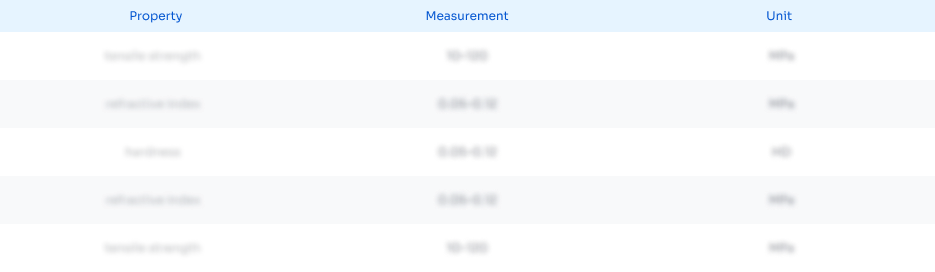
Abstract
Description
Claims
Application Information
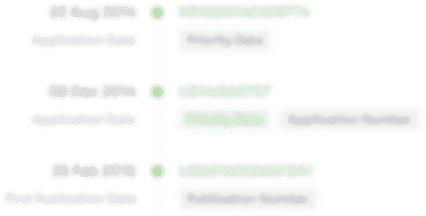
- R&D Engineer
- R&D Manager
- IP Professional
- Industry Leading Data Capabilities
- Powerful AI technology
- Patent DNA Extraction
Browse by: Latest US Patents, China's latest patents, Technical Efficacy Thesaurus, Application Domain, Technology Topic, Popular Technical Reports.
© 2024 PatSnap. All rights reserved.Legal|Privacy policy|Modern Slavery Act Transparency Statement|Sitemap|About US| Contact US: help@patsnap.com