Electromechanical equipment few-sample degradation trend prediction method of unsupervised meta-learning network
A technology of unsupervised learning and electromechanical equipment, applied in the direction of neural learning methods, prediction, biological neural network models, etc., can solve problems such as difficult labeling of historical data, and achieve the effect of significant generalization ability
- Summary
- Abstract
- Description
- Claims
- Application Information
AI Technical Summary
Problems solved by technology
Method used
Image
Examples
Embodiment Construction
[0028] The technical solution of the present application will be described in detail below in conjunction with the accompanying drawings.
[0029] figure 1 It is a flow chart of the method for predicting the degradation trend of electromechanical equipment with few samples in the unsupervised meta-learning network described in this application. As a specific embodiment, this application includes the following steps:
[0030] S1: collecting the vibration signal of the electromechanical equipment through the piezoelectric accelerometer, and preprocessing the vibration signal.
[0031] In this example, piezoelectric accelerometers are used to collect and analyze the vibration signals of two sets of petrochemical pumps when they run to failure. Among them, P1021B belongs to the distillation equipment, and P2209C belongs to the catalytic device. Their original signals and operating conditions can be found in figure 2 and Table 1. Then preliminarily perform frequency-domain nois...
PUM
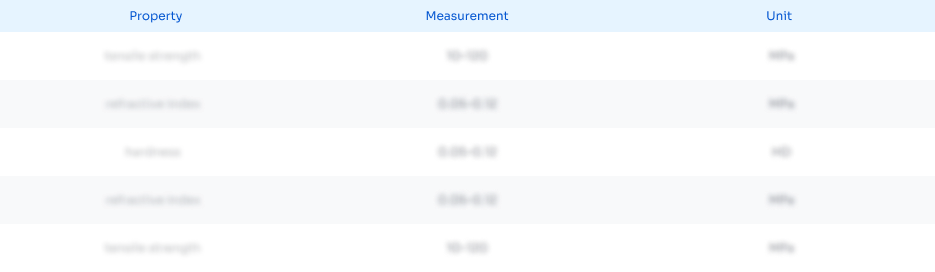
Abstract
Description
Claims
Application Information
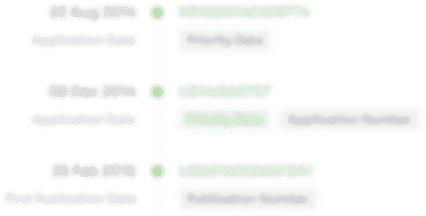
- R&D Engineer
- R&D Manager
- IP Professional
- Industry Leading Data Capabilities
- Powerful AI technology
- Patent DNA Extraction
Browse by: Latest US Patents, China's latest patents, Technical Efficacy Thesaurus, Application Domain, Technology Topic, Popular Technical Reports.
© 2024 PatSnap. All rights reserved.Legal|Privacy policy|Modern Slavery Act Transparency Statement|Sitemap|About US| Contact US: help@patsnap.com