Multi-low-level feature fusion retinal vessel segmentation method
A technology of retinal blood vessels and low-level features, applied in the field of retinal blood vessel segmentation with multi-low-level feature fusion, can solve the problems of wasting computing power, limited segmentation effect, low efficiency, etc., and achieve the effect of high classification accuracy
- Summary
- Abstract
- Description
- Claims
- Application Information
AI Technical Summary
Problems solved by technology
Method used
Image
Examples
Embodiment 1
[0037] Such as figure 1 As shown, this embodiment provides a retinal blood vessel segmentation method with multi-low-level feature fusion, including steps:
[0038] Step S1. Obtain the original fundus image set, and extract several low-level feature images respectively;
[0039] Step S2. Stacking the several low-level feature images together to form a feature vector containing background and blood vessel features;
[0040] Step S3. Acquire a manually marked fundus image set, and use the feature vector as an input for training the retinal vessel segmentation model to complete the model training;
[0041] Step S4. After the fundus image to be segmented is processed in the above steps S1 and S2, it is input into the trained retinal blood vessel segmentation model to obtain a segmented image containing only blood vessels and background.
[0042] It should be understood that the fundus image contains various features, but not all features are helpful to improve the performance of...
Embodiment 2
[0045] This embodiment is developed on the basis of the above-mentioned embodiment 1. This embodiment provides a specific example of extracting low-level feature images, including: extracting several low-level feature images in step S1 includes extracting color feature images; Color profile images include:
[0046] Green channel feature map F in RGB color space Green ; red and orange mask F in HSV color space HSV .
[0047] It should be understood that the acquired fundus image is a color image, which includes red, green and blue channel features. For the retinal vessel image, the color fundus image shows the strongest contrast in the image separated by the green channel, so this embodiment selects the green feature map as an important low-level feature.
[0048] On the other hand, the HSV color space includes black, white, red, orange, yellow, green, cyan, blue, and purple, where, in the red space, the large blood vessels of fundus images are displayed more clearly, while ...
Embodiment 3
[0050] This embodiment is developed on the basis of the above-mentioned embodiment 1. This embodiment provides another specific example of extracting low-level feature images, including: extracting several low-level feature images as described in step S1 including the brightness image F Lum ; The brightness image F Lum The extraction formula is:
[0051] f Lum =0.299*R+0.587*G+0.114*B; wherein, R, G, and B are the red, green, and blue channel feature images of the fundus image in RGB color space, respectively. It should be understood that in the fundus image, blood vessels in each region have different brightness values from the background, and different thresholds can effectively separate the blood vessels from the background. Therefore, this embodiment considers the brightness value as a feature. In the color retinal vascular images, the brightness of the blood vessels and the background in different regions are obviously different. Moreover, as described in the above-m...
PUM
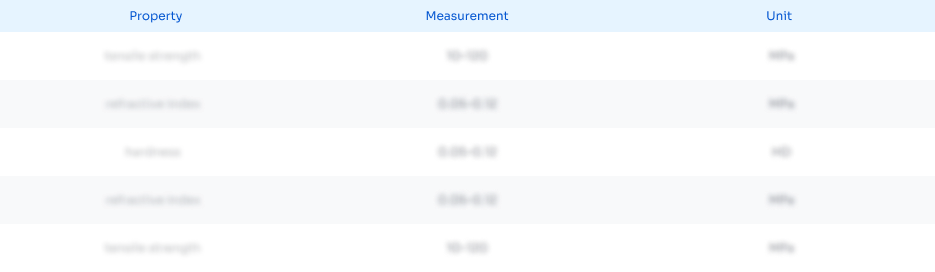
Abstract
Description
Claims
Application Information
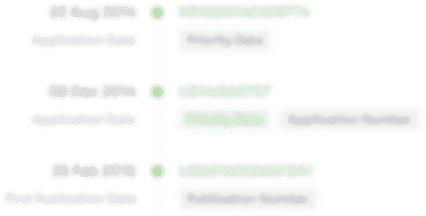
- Generate Ideas
- Intellectual Property
- Life Sciences
- Materials
- Tech Scout
- Unparalleled Data Quality
- Higher Quality Content
- 60% Fewer Hallucinations
Browse by: Latest US Patents, China's latest patents, Technical Efficacy Thesaurus, Application Domain, Technology Topic, Popular Technical Reports.
© 2025 PatSnap. All rights reserved.Legal|Privacy policy|Modern Slavery Act Transparency Statement|Sitemap|About US| Contact US: help@patsnap.com